本文侧重于如何从依据理论指导实践,在不适用任何第三方库的情况下实现ErasuceCode算法。本文仅会对用到的理论做简单的解释并标记引用,不会做详细解释。因此阅读本文前请熟读文献[1]第四章内容。
本文代码存储于https://github.com/zhengchenyu/SimpleErasureCode, SimpleErasureCode只是为了便于方便理解,为了保持与文章保持一致,因此没有优化。部分关键流程文章中会有链接到对应的代码。
EC算法在存储领域和通信领域都有广泛的应用。在分布式存储领域,为了避免机器宕机,需要存储3份冗余副本,这3台机器最多允许2台宕机。如果使用EC RS-6-3的话,可以实现使用9个副本冗余6份数据,这9台机器最多允许3台宕机,而且存储量却减少了一半。分布式存储使用EC在几乎不降低可用性的前提下,降低了冗余副本数,大大节约了存储资源。
实现EC算法是检验是否理解EC算法的重要手段。对EC算法的理解对工程上也有很大的帮助,笔者实现EC算法的初衷也是为了解决HADOOP-19180提出的问题。
1 算法概述
本文以RS-6-3算法为例, 存在6个数据块和3个校验块。EC算法的两个基本问题:
- 如何通过数据块生成校验块?
- 如何通过部分数据块和校验块恢复丢失的数据块?
1.1 生成校验块
生成校验块即编码过程(encode)。对6个数据块依次取出byte, 即d0,d1,d2,d3,d4,d5。如下图所示,用编码矩阵(encodeMatrix)乘以对应的数据块,即得到原有的数据d0,d1,d2,d3,d4,d5和对应的校验字节c0,c1,c2(编码过程)。对数据块的所有字节依次执行上述的操作就得到了校验块。
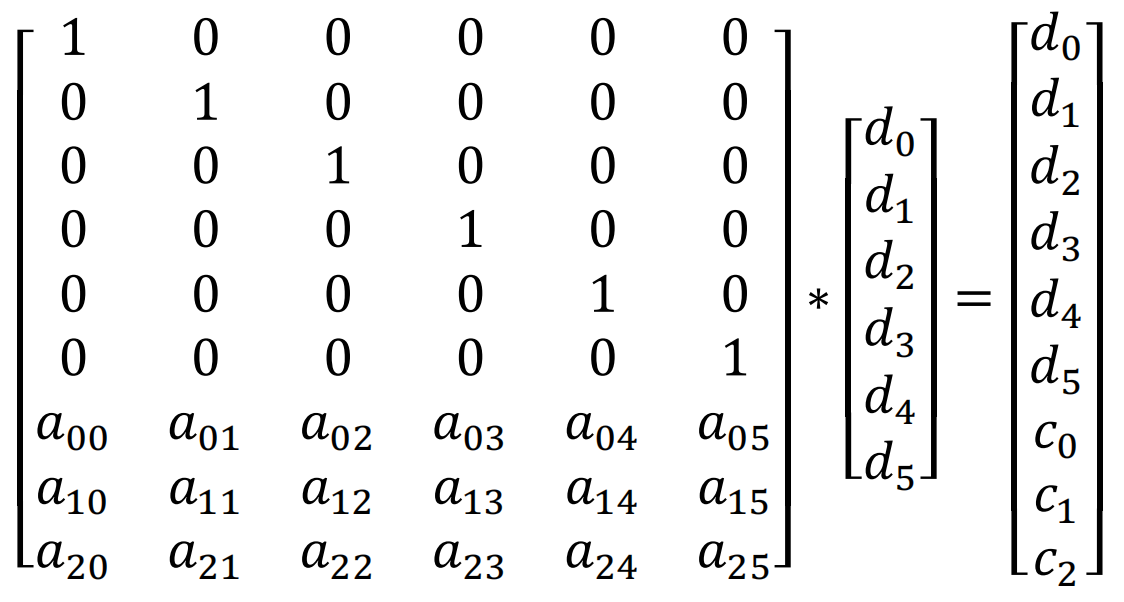
1.2 恢复数据块
恢复数据块的过程即解码过程(decode)。假如d2,d3,d4丢失了,我们需要通过d0,d1,d5,c0,c1,c2恢复d2,d3,d4。在上面公式中删除对应的行,公式中用d2?,d3?,d4?表示数据块丢失。有如下公式:
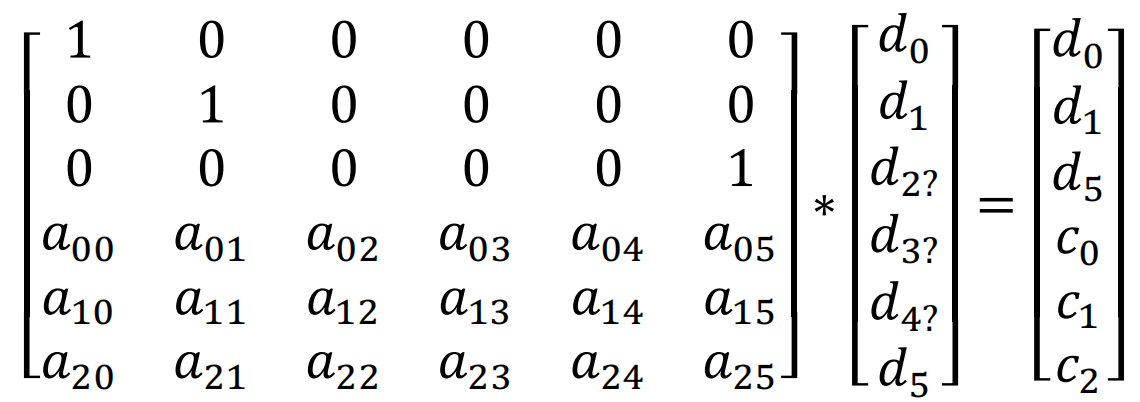
对上面的公式两侧都乘以裁剪后的矩阵的逆矩阵,这个逆矩阵即解码矩阵(decodeMatrix)。可以得到如下的公式:
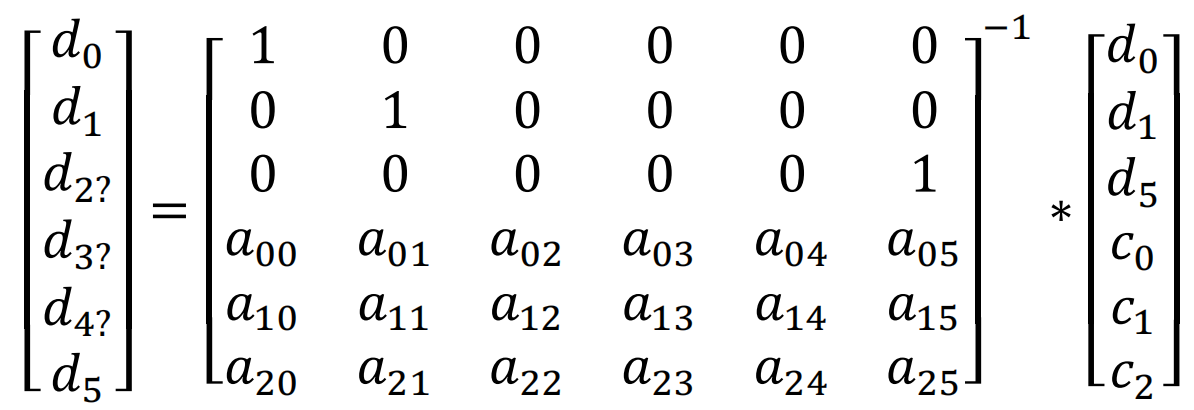
得到解码矩阵后其实计算过程与编码类似,只是输入为为d0,d1,d5,c0,c1,c2,输出为d0,d1,d2,d3,d4,d5。
2 关于矩阵
上一章节已经介绍了EC编解码的基本过程,但工程实现上让然会有一些问题需要解决。这一小节主要介绍一下关于矩阵方面的问题。
2.1 如何选择矩阵
根据第一小节的分析,编码矩阵是一个9 * 6的矩阵。而解码矩阵是在编码矩阵的基础上删除任意三行,然后再求逆的。因此我们定义编码矩阵的时候要保证,对于这个9 * 6的编码矩阵,任意删除三行得到的6 * 6的矩阵是一个可逆矩阵。
为了便于计算,编码矩阵的上半部分使用的是6 * 6 的单位矩阵,单位矩阵是可逆的,是满足条件的。接下来就是给下半部分的3 * 6的找到合理的矩阵。本文使用的是范德蒙矩阵。
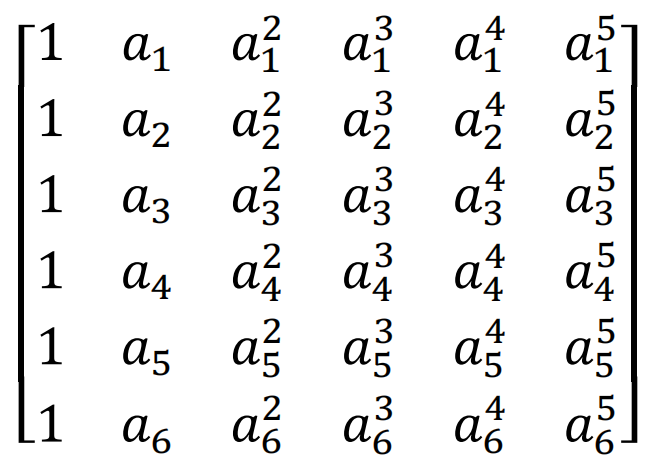
由于MathType不支持新版Mac, 而且markdown经常因为调整不好乱码,这里的共识还是贴图吧…
如下为范德蒙矩阵的行列式:
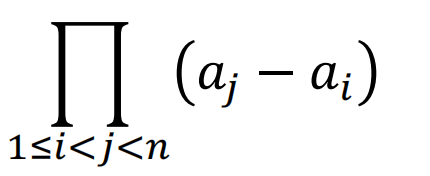
只要ai各不相等且不为0,则范德蒙矩阵一定可逆,意味着任意挑出3行向量,一定是线性无关的。那我们就挑出前三行,令a1=1,a2=2,a3=3,便可以得到如下编码矩阵:
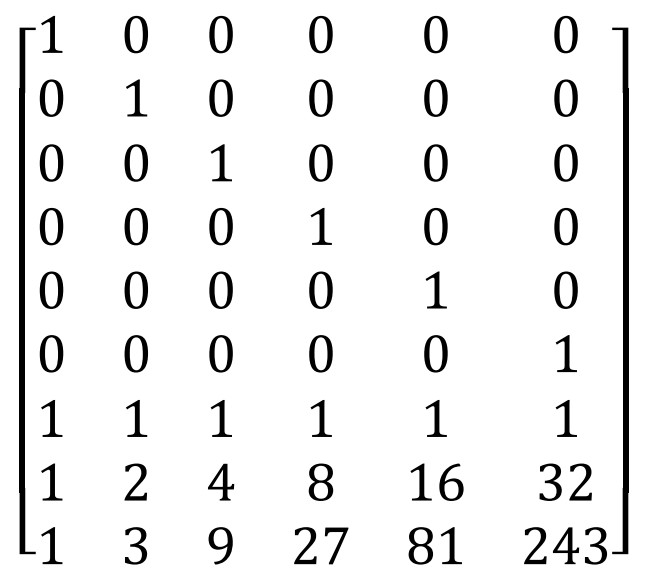
前面已经说明了前6行向量是互为线性无关,后3行向量也是互为线性无关的。如果在前6行中选取n行,在后三行中去6-n行,那么这6行向量还是线性无关的吗?假设n为5,由于后三行的没有元素为0,因此肯定是缺一个维度来保证线性相关。如果n为4和3也是同样的道理。因此这个9 * 6的矩阵随意挑出6行组成的6 * 6阶矩阵一定是可逆的。
2.2 高斯消元求逆
本文使用了容易理解的高斯消元法求逆(inverse)。假设当前6 * 6矩阵为A, 在右侧再拼接6 * 6的单位矩阵E,得到矩阵[A | E]。如果使用A-1乘以这个矩阵,会得到[E|A-1]。这样我们只需将A矩阵转化为单元矩阵,E矩阵自然就变成了A-1。(高斯消元求逆)
对每一行行依次执行如下的过程:
- (1) 对第i行, 找到第i行第i列的值。(Step1)
- (2) 然后计算该值的乘法逆元。然后让该行的每个元素均乘以这个乘法逆元。(Step2)
- (3) 对其他行j,使用第i行经过线性变换将地地j行第i列的值消为0。
经过这三步变换为,对于第i列,有且只有地i行的数据为1,其他均为0。对每一行均执行如下操作,左侧变得到了单位矩阵,右侧的结果也即A-1。
对于有理数域中,乘以乘法逆元即除法,加上加法逆元即减法。这样描述对伽罗华域中的数学运算的描述更准确。
对于第0行计算的过程如下,其他行同理。
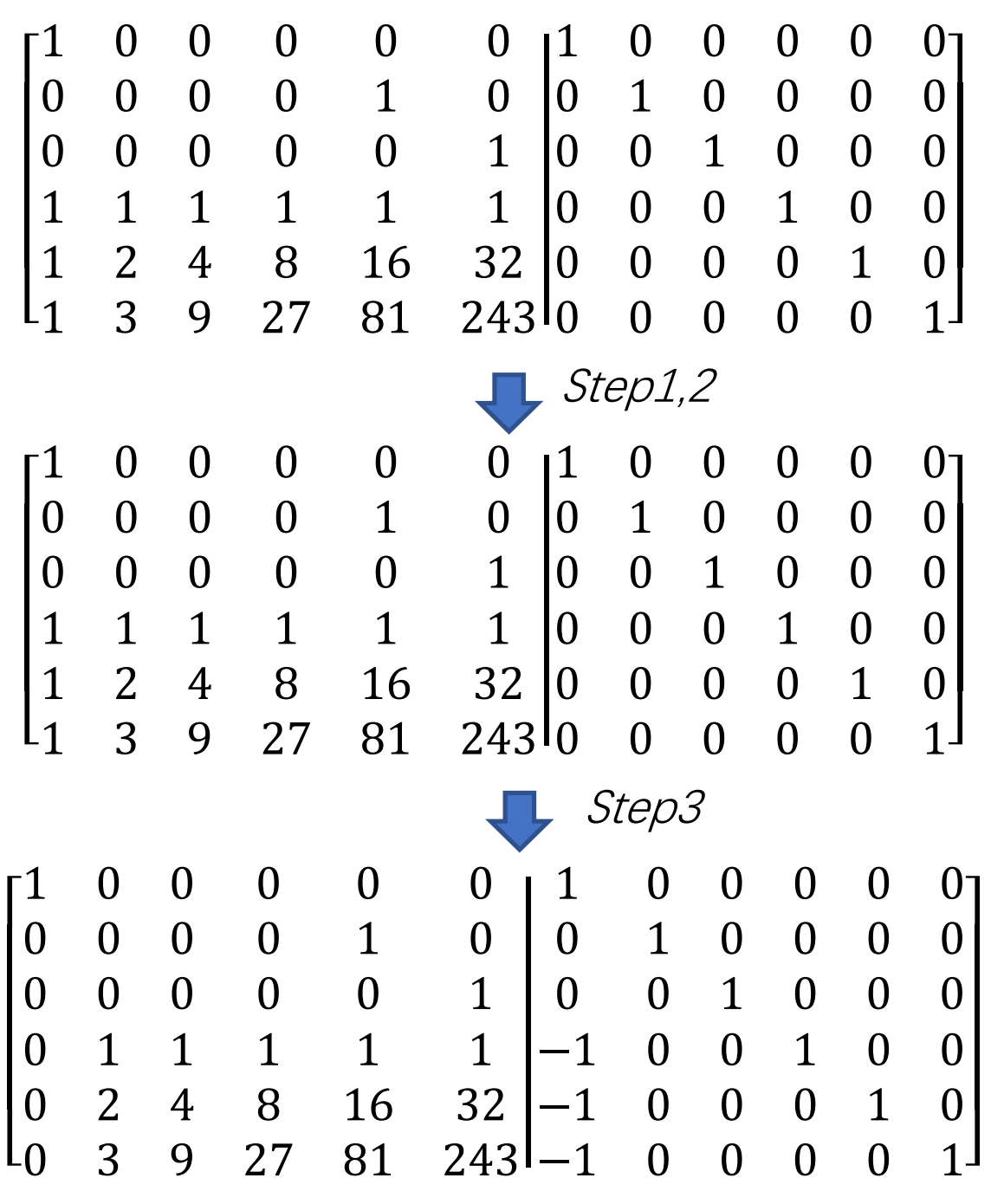
还有一种特殊的情况,譬如上述矩阵如果处理第2行。第2行和第2列的元素值为0,0是没有乘法逆元的。所以这时候就需要找到第2行下面的行中第2列不为0的情况,把该行加到第2行上,这样可以保证算法可运行(setup for step1)。其实移位可能更高效,但是为了便于理解,采用这样的方式。
3 伽罗华域
矩阵运算还存在一个问题,数据都是byte存储的,是一个0-255的数值。经过有理数运算后,数值是很容易越界的。因此我们需要一套新的计算体系同时满足以下两个条件:
- 该算法体系的值和计算得到的值只能是在有限的集合范围内,即不越界。
- 可以通过有限的运算恢复得到原有值,即可解码。
这就需要使用伽罗华有限域。伽罗华有限域可以保证任何计算得到的结果均在有限集合内,这满足了不越界的要求。另外伽罗华有限域的运算都有对应的逆运算。譬如对a,如果a乘以b,再乘以b的加法逆元,结果仍然是a。这满足了可解码的要求。
这里为了便于理解先简单的介绍GF(7),然后再解释实际使用的GF(28)。
本章并没有详细的证明。譬如将7扩张到无穷大的质数的时候,如何证明相应的定理为什么成立。事实上笔者也不知道,参考文献上也没有严谨的证明。也只是简单的说明了合数不能作为模数。但对于工程上有限的集合内,很容易通过穷举法来证明算法的可行性。
3.1 GF(7)
首先给出如下定义:
- 加法逆元: 给定x,如果存在x’,使得x+x’=x’+x=0,则称x’是x的加法逆元。
- 乘法逆元: 给定x,如果存在x’,使得x * x’=x’ * x=e,则称x’为x的乘法逆元。其中e为该群的单位元。对于GF(7),e为1。
我们定义一种新的运算,即模7运算。对于加法和乘法运算,我们会将结果然后模7。譬如, 8 + 3 = 11 mod 7 = 4。8 * 3 = 24 mod 7 = 3。
对于加法逆元和乘法逆元,根据定义我们可以穷举加法和乘法计算,根据结果得到对应的逆元。譬如, 4 + 3 = 7 mod 7 = 0,我们就说4和3互为加法逆元。2 * 4 = 8 mod 7 = 1,我们就说2和4互为乘法逆元。
下表穷举了GF7的所有加法和乘法运算,根据码表也得到了GF(7)下所有元素对应的加法逆元和乘法逆元。
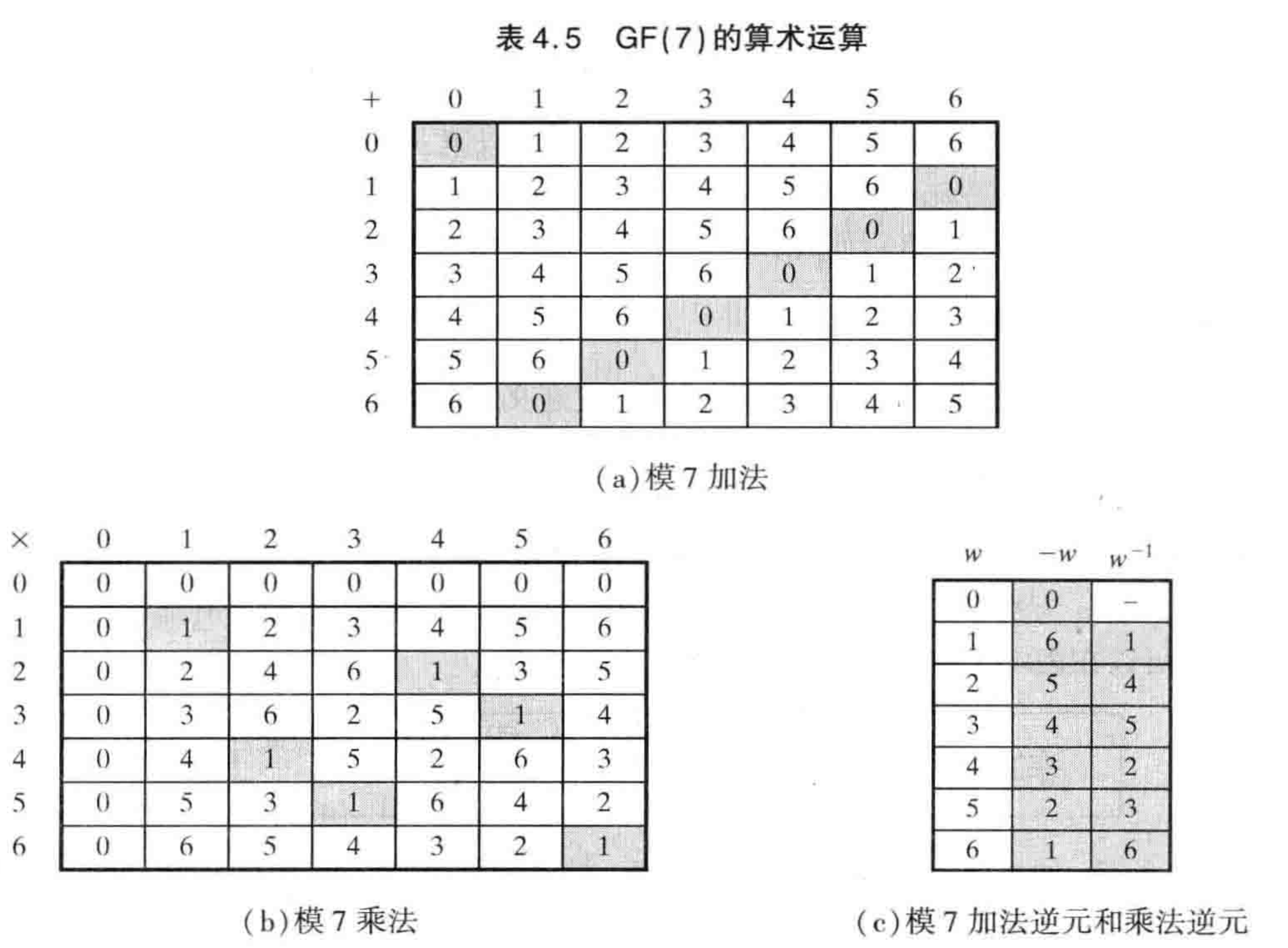
来看下是否满足我们的要求,不越界在定义上就满足了。可解码的特点,我们随机指定数组假设为5,用5乘以3在乘以3的乘法逆元5, 得到的值为5 * 3 * 5 = 75 mod 1 = 5。事实上根据乘法交换律很容易证明这个。对于加法逆元的解码同理。
3.2 GF(28)
3.2.1 基本原理
实际的数字存储的值是0-255, 那么是否意味着我们可以直接使用GF(256)呢? 答案是不可以的。因为256是合数,譬如16 * 16 = 256 mod 256 = 0,那么16就不存在乘法逆元,也就难以进行边界吗。
因此需要引入多项式的运算,且要求同指数幂下遵循GF(2)。模数为不可约多项式。对于不可约多项式a, 无法找到两个不为1的多项式b和c使得b * c = a。可以使用穷举法得到每个多项式的加法逆元和乘法逆元。事实上只要集合内的每个元素都有1对1对应的乘法逆元,就可以满足我们的要求。文献1的表4.6也通过穷举证明了GF(23)的有效性。
多项式的计算与对byte的编码有什么关系呢?由于多项式的同指数幂是GF(2),也就意味着对于多项式a0 + a1x + a2x2 + a3x3 + a4x4 + a5x5 + a6x6 + a7x7 ,ai 为0和1。如果a0为第0位,a1为第1位,依次类推,这组系数就是一个byte。这样就把要存储的byte与多项式运算结合了。
3.2.2 计算
本章主要介绍如何计算GF(28)。对于加法和乘法,我们直接使用多项式运算。对于加法逆元和乘法逆元,我们穷举加法和乘法运算,然后通过码表来得到加法和乘法逆元。
本文的算法的不可约多项式为x8 + x4+ x3 + 1。
f(x) = x6 + x4+ x2 + x + 1, g(x) = x7 + x + 1
对于加法, 指数幂执行GF(2)运算,G(2)运算实际上就是异或。得到f(x)+g(x)= x7 + x6 +x4+ x2 + 1。实际可以理解为f(x)对应的二进制0b01010111与g(x)对应的二进制0b10000011进行按位的异或计算。(GF(28))
对于乘法, 先考虑h(x) = a0 + a1x + a2x2 + a3x3 + a4x4 + a5x5 + a6x6 + a7x7, 计算 h(x) * x = (a0x + a1x2 + a2x3 + a3x4 + a4x5 + a5x6 + a6x7 + a7x8 ) mod (x8 + x4+ x3 + x + 1)。存在两种情况
- (1) a7 等于 0
那么该式已经不可约,因此h(x) * x= a0x + a1x2 + a2x3 + a3x4 + a4x5 + a5x6 + a6x7。也就意味着[a7a6a5a4a3a2a1a0] * [00000010] = [a6a5a4a3a2a1a00],即向左移动一位。(a7 等于 0) - (1) a7 不等于0
h(x) * x = (a0x + a1x2 + a2x3 + a3x4 + a4x5 + a5x6 + a6x7 + x8) mod (x8 + x4+ x3 +x+ 1) = a0x + a1x2 + a2x3 + a3x4 + a4x5 + a5x6 + a6x7 + x8 - x8 - x4 - x3 -1 = 1 + (a0+1)x + a1x2 + (a2+1)x3 + (a3+1)x4 + a4x5 + a5x6 + a6x7。以为意味着[a7a6a5a4a3a2a1a0] * [00000010] = [a6a5a4a3a2a1a00] ^ [00011011]。即左移一位后,在于不包含最高位系统的字节进行异或。(a7 不等于 0)
这里减法为加法逆元,对于GF(2),加法逆元就是自己。
f(x) * g(x) = ((x6 + x4+ x2 + x + 1) * ( x7 + x + 1))。我们可以把他分解为一下三个运算之和。
- (1) (x6 + x4+ x2 + x + 1) * x7
- (2) (x6 + x4+ x2 + x + 1) * x
- (3) (x6 + x4+ x2 + x + 1) * 1
对于(2)在前面已经介绍了,对于(3)实质上不用计算。对于(1), 我们可以使用(2)的计算方法进行递归计算(fxxn)。然后分别计算的三个值进行相加便得到了最终的结果(_mul)。
至此,ErasureCode算法的所有问题都已经得到了解释。本文以自顶向下的方式描述了如何从理论到实践来实现ErasureCode算法。完整的代码见SimpleErasureCode。
参考文献:
- [1]William Stallings. 密码编码学与网络安全 原理与实践 第六版[M] 第四章
- [2]https://drmingdrmer.github.io/tech/distributed/2017/02/01/ec.html
RSS-远程Merge的设计
1 默认的shuffle
注: 第一节简单介绍了主流框架默认的shuffle的原理,目的为了那里会使用本地磁盘,从而设计远程Merge。如果足够了解,可以忽略这部分内容。
我们依次对MapReduce, Tez, Spark的shuffle进行分析。
1.1 MapReduce
Map将Record写入到内存,当内存超过阈值的时候,会将内存的数据spill到磁盘文件中,按照partitionid+key的顺序依次将Record写入到磁盘文件。当Map处理完所有Record后, 会将当前内存中的数据spill到磁盘文件。然后读取所有的spill到磁盘的文件,并按照partitionid+key的顺序进行merge,得到排序的Records。
注: 按照partitionid排序的目的是Reduce端从Map端获取的数据的时候,尽可能顺序读。对于MR、Tez、Spark, 无论是否排序,只要有分区,都需要按照partitionid进行排序。
Reduce端会从Map端以远程或本地的方式拉取对应分区的Records,称之为MapOutput。正常情况下会直接使用内存,如果内存超过阈值会将这些Records写入到磁盘。然后Reduce端会对所有MapOutput使用最小堆K路归并排序进行一些Merge操作,得到全局排序的Reccords。Merge的过程中,可能会因为内存超过阈值,会将临时的结果spill到磁盘。另外如果spill到磁盘的文件过多,也会触发额外的merge。
1.2 Tez
Tez的情况略微复杂。Tez分为ordered io和unordered io。
ordered io与MapReduce相同,不再展开分析。
unordered io一般用于hashjoin等不需要key进行排序的情况。非排序的io采用来之即用方案。Map直接将Record写入文件或者通过缓存在写入文件。Reduce端也是读数据的时候也是读之即用。
1.3 Spark
spark的shuffle更复杂,也更合理。部分任务是不需要sort和combine的,因此spark用户可以需求决定shuffle的逻辑。
1.3.1 Shuffle写操作
写shuffle数据的时候,支持三种writer:
- (1) BypassMergeSortShuffleWriter
为每个partition都生成一个临时文件。在写Record的时候,找到对应的分区直接写入对应的临时文件。然后当所有数据都处理完成后,将这些临时的文件按照分区的顺序依次写入到一个最终的文件,并删除临时文件。
- (2) UnsafeShuffleWriter
UnsafeShuffleWriter主要通过ShuffleExternalSorter来实现具体的逻辑。当写Record的时候,直接序列化操作,并将序列化的字节拷贝到申请的内存中。同时也会将Record的地址和分区记录到内存中(inMemSorter)。
当内存的Record达到阈值的时候,会进行spill操作。根据内存(inMemSorter)中的信息,我们很容易得到一个按照分区排序的Record,并写到文件中。
当处理完所有Record,我们会把当前内存中的Records spill到文件中。最后对所有spilled文件进行一次聚合。由于之前spilled的文件已经是按照分区排序的,所以我们可以按照分区的顺序依次将所有spill的文件的对应自己拷贝到最终文件。这样得到的最终文件即为分区排序的文件。
- (3) SortShuffleWriter
SortShuffleWriter主要通过ExternalSorter来实现具体逻辑。ExternalSorter根据用户的需求决定是否combine和sort。
当写Record的时候,会直接插入到内存中。如果需要combine,内存架构是map,否则是buffer。
如果当前评估内存大于阈值会触发spill操作。spill操作的时候,会将Record,然后spill到磁盘。这个过程是需要进行排序的。而具体的比较器会根据用户需求的不同使用不同的值。如果设置了keyordering会按照key进行排序。如果没有设置keyordering,但是设置了aggregator(即combine),则按照key的hashcode进行排序,这样保证相同的key组织一起,便于combine操作。如果keyordering和aggregator都没有设置,则会按照partiton进行排序。
所有Record都写完时,需要读取spill的文件,并合并成一个全局有序的文件。
三种writer的比较
writer | 优点 | 缺点 | 场景 |
---|---|---|---|
BypassMergeSortShuffleWriter | (1) 只经过一次序列化。 (2) 采用类hashmap的数据结构,插入数据快。 |
(1) 不支持combine (2) 每个分区都要对应生成一个临时文件,会产生过多的临时文件。 |
适合分区数较少(默认小于等于200)且没有combine的的情况. |
UnsafeShuffleWriter | (1) 只经过一次序列化。 (2) spill到磁盘的文件数目有限,不再基于分区数,可以支持更大的分区。 |
(1) 不支持combine (2) 写入顺序Record顺序会打乱,要求supportsRelocationOfSerializedObjects。 |
适用于没有combine的情况,且支持supportsRelocationOfSerializedObjects,并且支持最大支持分区数为16777216。 |
SortShuffleWriter | (1) 支持combine (2) 适合于所有场景 (3) spill到磁盘的文件数目有限 |
(1) 需要进行多次序列化 | 适用于所有场景。 |
1.3.2 shuffle读
当前只有BlockStoreShuffleReader一个实现。实现与MapReduce类似。
Reduce端会从Map端以远程或本地的方式拉取对应分区的Records。正常情况下会直接写到内存中,但如果要获取的block大小超过阈值则会使用磁盘。
然后会根据用户的需求决定是否进行combine或sort,最终形成一个用户要求的record iterator。
combine和sort分别使用了ExternalAppendOnlyMap和ExternalSorter,当内存超过阈值后,会将数据spill到本地磁盘中。
1.4 总结
(1) 关于各个框架语义
对于MapReduce和Tez的ordered io, 实质就是spark的排序的特例。对于Tez的unordered io,实质上就是spark的非排序的特例。实质各个框架上语义是相同的, spark更加泛化。
(2) 哪里会产生本地磁盘文件?
分析三种计算框架后,我们得知如下过程会使用磁盘:
- (1) Map由于内存超越阈值,可能会产生中间的临时文件。
- (2) Map端最终必然会产生磁盘文件,用于提供shuffle服务。
- (3) Reduce拉取records时候,可能因为超越阈值,产生磁盘文件。
- (4) Reduce端Merge的时候,可能会产生临时的磁盘文件,用于全局排序。
而事实上, uniffle已经解决(1), (2)。对于(3), 如果有效的调整参数,是很难产生磁盘文件的。事实上只有(4)是本文需要讨论的。
2 方案的选择
为了解决在Reduce端Merge可能会spill到磁盘的问题,主要有两个方案:
- (1) Shuffle Server端进行Merge
- (2) Reduce端按需Merge
2.1 方案1: ShuffleServer端进行Merge
将Reduce的Merge过程移到ShuffleServer端,ShuffleServer会对Map端发来的局部排序后的Records进行Merge,合并成一个全局排序的Records序列。Reduce端直接按照哦Records序列的顺序读取。
- 优点: 不需要过多内存和网络RPC。
- 缺点: Shuffle Server端需要解析Key, Value和Comparator。Shuffle端不能combine。
2.2 方案2: Reduce端按需Merge
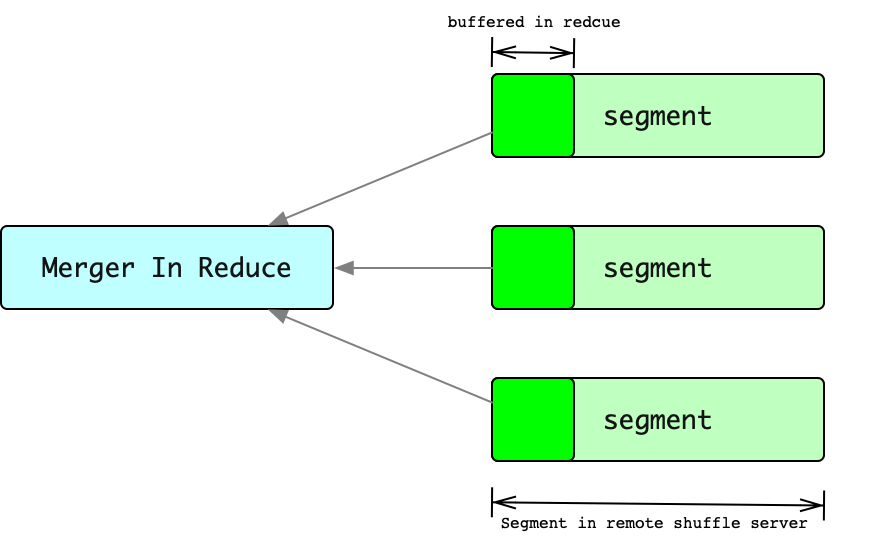
这里的segment是指排序后record集合,可以理解为record已经按照key排序后的block。
- 优点: Shuffle Server不需要做额外的任何事情。
- 缺点: 过多的RPC。
本文选择方案1,接下来的内容主要针对于方案1进行讨论。
3 需求分析
3.1 哪类任务需要远程merge?
当前uniffle的map端操作已经不再需要磁盘操作。本文主要考虑reduce端的情况。主要分如下几种情况:
- (1) 对于spark的非排序且非聚集、tez unordered io,Record是来之即用的,不需要有任何的全局的聚合和排序操作,只需要非常少的内存。当前版本的uniffle在内存设置合理的情况下是不会使用磁盘的。使用当前uniffle的方案即可。本文不会讨论这方面的内容。
- (2) 对于spark的排序或聚集任务、tez ordered io、mapreduce,由于需要全局排序或聚集,内存可能不够用,可能会将Record spill到磁盘。本文主要讨论这种情况。
综上可知,remote merge仅用于需要排序或聚集的shuffle。
3.2 ShuffleServer如何进行排序?
对于排序类的操作,一般会在Map进行排序得到一组局部排序的记录,这里称之为segment。然后Reduce会获取所有的segment, 并进行归并,Spark, MR, Tez都是用了最小堆K路归并排序。远程排序依旧可以使用这种方式。
BufferManager和FlushManager维护着block在内存和磁盘中的信息。我们只需要在需要在ShuffleServer中新增MergeManager,并将同一个Shuffle下的block进行Merge,得到全局排序的Records即可。
在ShuffleServer端引入排序后产生一个副作用: 即需要将该Shuffle的KeyClass和ValueClass以及KeyComparator传递给ShuffleServer。
3.3 ShuffleServer禁止combine
Combine一般都是用户自定义的操作,因此禁止ShuffleServer端进行Combine操作。
4 架构设计
4.1 RemoteMerge的基本流程

下面介绍一下Remote Merge的流程:
- (1) 注册
AM/Driver调用registerShuffle方法,会额外注册keyClass, valueClass和keyComparator. 这些信息主要用于ShuffleServer在合并的时候对Record进行解析和排序。 - (2) sendShuffleData
sendShuffleData逻辑与现有的RSS任务基本保持一致。唯一区别是使用统一的序列化器和反序列化器,这样可以保证无论是哪一种框架,ShuffleServer都可以正常的解析Record. - (3) buffer and flush
shuffle server端会将数据存在缓存中,或者通过flush manager缓存到本地文件系统或远程文件系统。这里还是复用原来的ShuffleServer的逻辑。 - (4) reportUniqueBlocks
提供了一个新的API, 即reportUniqueBlocks。Reduce端会根据map产生的block进行去重,然后将得到有效block集合通过reportUniqueBlocks发送给ShuffleServer。ShuffleServer收到有效的blocks集合后,会触发Remote Merge。Remote Merge的结果会像普通的block一样写入到bufferPool, 避免的时候会flush到磁盘中。RemoteMerge产生的结果即为普通的block,但是为了方便说明,这里称之为merged block。merged block记录的是按照key排序后的结果,因此读取merged block的时候,需要按照blockid的顺序依次递增读取。 - (5) getSortedShuffleData
Reduce会按照block序号的顺序读取merged block,然后根据一定的条件选择何时为reduce计算使用。
4.2 从Record的视角分析流程
我们可以WordCount为例子解释Record在整个过程中的流转。本例子中有两个分区以及一个Reduce,即一个Reduce处理两个分区的数据。
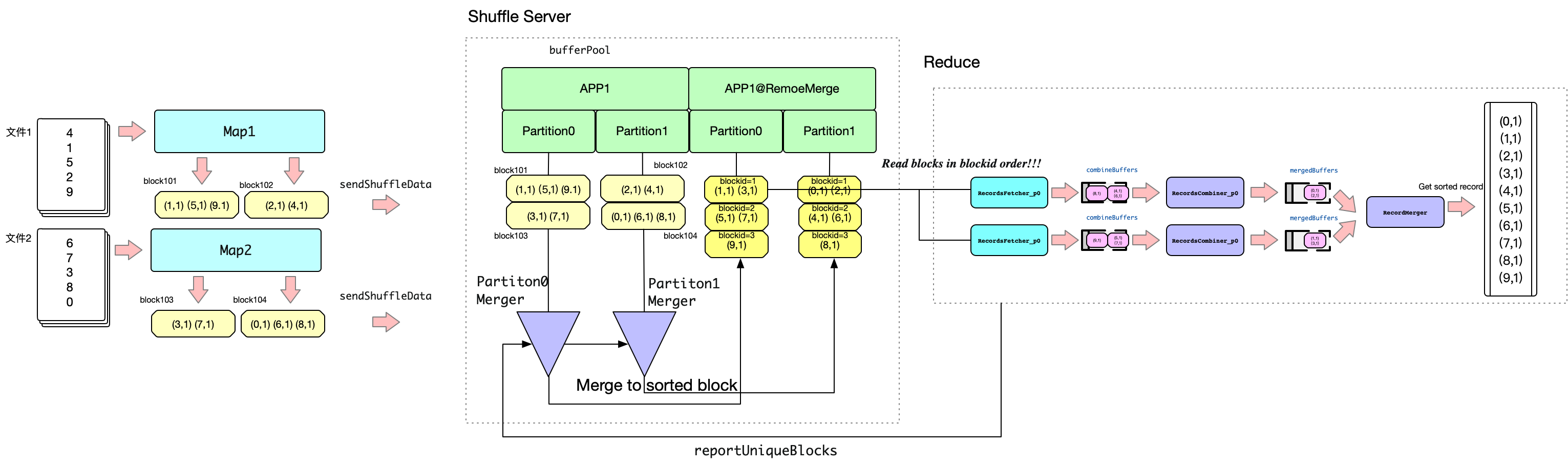
- (1) MAP端
Map端处理文档数据后,会进行排序。对于Map1, 由于存在两个分区,以奇数为key的record会写入到block101中,以偶数为key的record会写入到block102中。Map2同理。注意这里block中的Record都是已经排序后的。 - (2) Map端发送数据
Map端通过sendShuffleData将block发送给ShuffleServer, ShuffleServer会将其存储到bufferPool中。
这里指的注意的是,在注册的时候会会注册APP1名字的app的同时,也会注册APP1@RemoteMerge的app,稍后会介绍它。 - (3) ShuffleServer端Merge
Reduce启动后,会调用reportUniqueBlocks汇报可用的block集合,同时触发ShuffleServer中对应的partition进行Merge。Merge的结果在这个分区下全局排序的Record集合。
然后的问题是Merge的结果存在那里?Merge过程是在内存中发生的,每当Merge一定数量的Record后,会将这些结果写到一个新的block中。为了与原来的appid区分,这里会将这组block放在一个以”@RemoteMerge”结尾的appid进行管理。这组新的block的blockid是从1开始递增的,而且是经过全局排序的。即每个block内部的record是排序的,blockid=1的records一定小于等于blockid=2的records。 - (4) Reduce端读
根据前面的分析,Reduce端只要读取以”@RemoteMerge“结尾的appid管理的block即可。Reduce读取block的时候从blockid=1的block开始,按照blockid顺序读取即可。我们知道Reduce进行计算的时候,是按照顺序计算的。由于我们在ShuffleServer端获取的数据已经是排序后的,所以每次只需要从ShuffleServer端获取少量的数据即可,这样就实现了从ShuffleServer端按需读取,大大降低了使用内存。
这里还存在两种特殊的情况,详细5.5
5 计划
5.1 统一序列化器
由于需要在ShuffleServer端进行Merge, 需要提取出独立于计算框架的统一序列化器。这里提炼出两类序列化器: (1) Writable (2) Kryo。Writable序列化用于处理org.apache.hadoop.io.Writable接口的类,用于MR和TEZ框架。Kryo可以序列化绝大多数的类,一般用于Spark框架。
5.2 RecordsFileWriter/RecordFileReader
提供关于处理Record的抽象方法
5.3 Merger
提供基础的Merger服务,对多个数据流按照key进行merge。采用最小堆K路归并排序,对已经进行局部排序的数据流进行归并排序。
5.4 MergeManager
用于在服务端对Records进行Merge。
5.5 RMRecordsReader
一般来讲Reduce端在读取数据的情况,直接发给下游计算即可。但是存在两种特殊的情况:
(1) 对于存在需要在Merge进行combine的情况,我们需要等待所有相同的key都达到后进行combine,然后再发给下游。
(2) 对于spark和tez, reduce端可以会读取多个分区的数据。因此我们需要对多个分区的数据在reduce端再进行一次merge,然后在发给下游。
RMRecordsReader是用于读取排序后数据的工具。大致的架构如下:

图中描述了单个Reduce处理两个分区的情况。RecordsFetcher线程会读取对应分区的block,然后解析成Records。然后发送到combineBuffers中。RecordCombiner从combineBuffer中读取Records,当某个key的所有records都收集完成,会进行combine操作。结果会发送给mergedBuffer。RecordMerge会获取所有mergedBuffer,然后在内存中再进行一次归并排序。最终得到全局排序的结果给下游使用。
5.6 框架适配
适配MR,Tez,Spark三种架构。
笔者已使用线上任务对MR和Tez进行了大规模压测。Spark目前仅进行了一些基础的examples的测试,仍需要大量测试。
5.7 隔离的classloader
对于不同版本的keyclass, valueclass以及comparatorclass, 使用隔离的classloader加载。
5 特别注意
- 不支持spark的javardd,因为spark javardd的类型会被擦除。
- 适当提高服务器的max open file的配置。因为合并的时候可能会长时间持有文件。
- 适当降低rss.server.buffer.capacity, 因为remote merge的过程需要更多的额外内存。
RSS-Remote Merge Design
1 Default shuffle
Note: The first chapter briefly introduces the principle of default shuffle, with the purpose of find where local disks are used, then design remote merge. If you know enough, you can ignore this part.
We will analyze the shuffle of MapReduce, Tez, and Spark in turn.
1.1 MapReduce
Map writes the record to the memory. When the memory exceeds the threshold, the memory data is spilled to the disk file, and the Record is written to the disk file in order of partitionid+key. After Map has processed all records, it will spill the data currently in memory to a disk file. Then read all the files spilled to the disk and merge them in the order of partitionid+key to get the sorted Records.
Note: The purpose of sorting according to partitionid is that when the Reduce side obtains the data from the Map side, it should be read as sequentially as possible. For MR, Tez, and Spark, regardless of whether they are sorted or not, as long as there are partitioned, they need to be sorted according to partitionid.
The reduce will pull the records of the corresponding partition remotely or locally from the Map, which is called MapOutput. Under normal circumstances, the memory will be used directly. If the memory exceeds the threshold, these records will be written to the disk. Then the reduce will perform merge operations on MapOutputs using minimum heap K-way merge sorting to obtain globally sorted records. During the Merge process, temporary results may be spilled to disk because the memory exceeds the threshold. In addition, if there are too many files spilled to disk, additional merges will be triggered.
1.2 Tez
There are two cases of tez: (1) ordered io (2) unordered io.
Ordered io is the same as MapReduce and so ignore it here.
Unordered io is generally used in hashjoin and other situations where keys are not required for sorting. Non-sorted io adopts a ready-to-use solution. Map writes the Record directly to the file or writes it to the file through cache. The Reduce side can also read and use it when reading data.
1.3 Spark
Spark’s shuffle is more complex and more reasonable. Some tasks do not require sort and combine, so spark users can determine the shuffle logic according to their needs.
1.3.1 Shuffle write operation
When writing shuffle data, three writers are supported:
- (1) BypassMergeSortShuffleWriter
A temporary file is generated for each partition. When writing record, find the corresponding partition and write it directly to the corresponding temporary file. Then when all data is processed, these temporary files are written to a final file in order of the partitions, and the temporary files are deleted.
- (2) UnsafeShuffleWriter
UnsafeShuffleWriter mainly implements specific logic through ShuffleExternalSorter. When writing a Record, the serialization operation is performed directly and the serialized bytes are copied to the requested memory. At the same time, the address and partition of the record will also be recorded into the memory (inMemSorter).
When the memory reaches the threshold, spill operation will be performed. Based on the information in memory (inMemSorter), we can easily get a Record sorted by partition and write it to a file.
When all Records are processed, we will spill the records currently in memory into the file. Finally, all spilled files are aggregated once. Since the previously spilled files have been sorted according to the partition, we can copy the corresponding copies of all the spilled files to the final file in the order of the partitions. The final file obtained in this way is the partition-sorted file.
- (3) SortShuffleWriter
SortShuffleWriter mainly implements specific logic through ExternalSorter. ExternalSorter decides whether to combine and sort based on the user’s needs.
When writing record, it will be inserted directly into memory. If combine is required, the memory architecture is map, otherwise it is buffer.
If the current evaluation memory is greater than the threshold, the spill operation will be triggered. During the spill operation, the Record will be spilled to the disk. This process requires sorting. The specific comparator will use different values according to different user needs. If keyordering is set, it will be sorted by key. If keyordering is not set, but aggregator (i.e. combine) is set, the keys are sorted according to the hashcode of key, thus ensuring that the same keys are organized together to facilitate combine operations. If neither keyordering nor aggregator is set, it will be sorted according to partition.
When all Records are written, the spill files need to be read and merged into a globally ordered file.
Comparison of three writers
writer | advantages | disadvantages | scene |
---|---|---|---|
BypassMergeSortShuffleWriter | (1) Only serialized once. (2) Using hashmap-like data structure, inserting data is fast. |
(1) Combine and sort are not supported (2) Each partition must generate a temporary file, which will generate too many temporary files. |
Suitable for situations where the number of partitions is small (default is less than or equal to 200) and there is no combine. |
UnsafeShuffleWriter | (1) Only serialized once. (2) The number of files spilled to disk is limited and is no longer based on the number of partitions, and can support larger partitions. |
(1) Combine, sort is not supported (2) The writing order Record order will be disrupted, and supportsRelocationOfSerializedObjects is required. |
Applicable to situations where combine does not exist, and supportsRelocationOfSerializedObjects is true, and the maximum number of supported partitions is 16777216. |
SortShuffleWriter | (1) Supports combine, sort (2) Suitable for all scenarios (3) The number of files spilled to disk is limited |
(1) Multiple serializations are required | Suitable for all scenarios. |
1.3.2 shuffle read
Currently there is only one implementation of BlockStoreShuffleReader. The implementation is similar to MapReduce.
The reduce will pull the records of the corresponding partition remotely or locally from the map. Under normal circumstances, it will be written directly to the memory, but if the block size to be obtained exceeds the threshold, will use disk.
Then it will be decided according to the user’s needs whether to combine or sort, and finally form a record iterator required by the user.
Combine and sort use ExternalAppendOnlyMap and ExternalSorter respectively. When the memory exceeds the threshold, the data will be spilled to the local disk.
1.4 Summary
(1) About the semantics of each framework
For MapReduce and the ordered io of Tez, it is a special case of spark sorting. For Tez’s unordered io, it is essentially a special case of spark’s non-sorting. In essence, the semantics of each framework are the same, and spark is more general.
(2) Where will generate local disk files?
After analyzing the three computing frameworks, we learned that the following processes will use disks:
- (1) Map may generate intermediate temporary files because the memory exceeds the threshold.
- (2) The map will eventually generate disk files to provide shuffle services.
- (3) When reduce pulls records, disk files may be generated because the threshold is exceeded.
- (4) When merging on the reduce side, temporary disk files may be generated for global sorting.
In fact, uniffle has solved (1), (2). For (3), if the parameters are adjusted effectively, it is difficult to generate disk files. In fact, only (4) needs to be discussed in this article.
2 Plans
In order to solve the problem that Merge on the Reduce side may spill to disk, there are two main solutions:
- (1) Merge on Shuffle Server
- (2) Reduce side Merge on demand
2.1 Option 1: Merge on ShuffleServer
Move the merge process of reduce to the ShuffleServer side. ShuffleServer will merge the locally sorted Records sent from the map side into a globally sorted records sequence. The reduce side reads directly in the order of the records sequence.
- Advantages: Does not require too much memory and network RPC.
- Disadvantages: Shuffle Server needs to parse Key, Value and Comparator. The Shuffle side cannot combine.
2.2 Option 2: On-demand Merge on the Reduce side
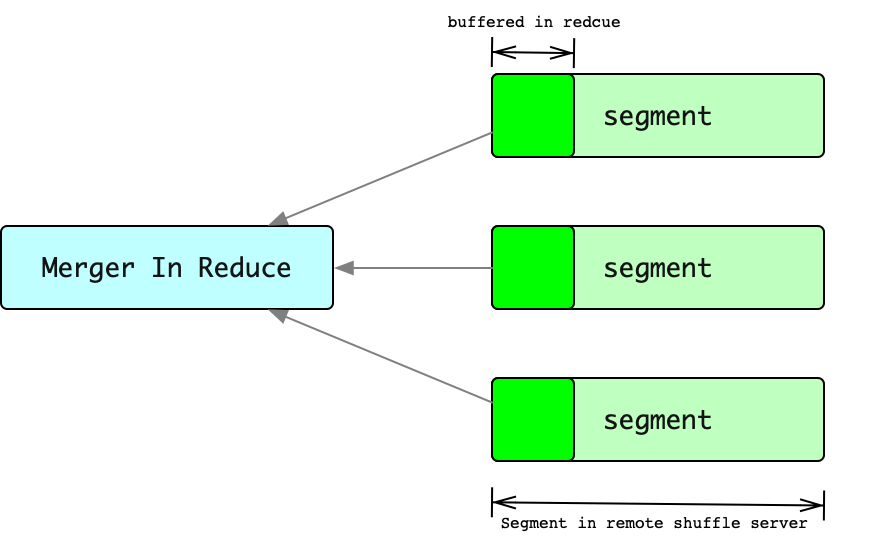
The segment here refers to the sorted record collection, which can be understood as the block in which the records have been sorted according to key.
- Advantages: Shuffle Server does not need to do anything extra.
- Disadvantages: Too many RPCs.
**This article chooses option 1, and the following content mainly discusses option 1. **
3 Demand analysis
3.1 What types of tasks require remote merge?
Currently, uniffle’s map-side no longer spill disk. This article mainly considers the situation on the reduce. Mainly divided into the following situations:
- (1) For spark’s non-sorted, non-aggregated, tez unordered io. It does not require any global aggregation and sorting operations, and only requires very little memory. The current version of uniffle will not use disk if related settings are reasonable. Just use the current uniffle solution. This article will not discuss this aspect.
- (2) For spark sorting or aggregation tasks, tez ordered io, mapreduce, due to the need for global sorting or aggregation, the memory may not be enough, and the record may be spilled to the disk. This article mainly discusses this situation.
**In summary, it can be seen that remote merge is only used for shuffles that require sorting or aggregation. **
3.2 How does ShuffleServer sort?
For sorting, map is generally sorted to obtain a set of partially sorted records, which is called segment here. Then reduce will obtain all segments and merge them. Spark, MR, and Tez all use minimum heap K-way merge sorting. This method can still be used for remote sorting.
BufferManager and FlushManager maintain block information in memory and disk. We only need to add MergeManager to ShuffleServer and merge the blocks under the same Shuffle to obtain globally sorted Records.
Introducing sorting on the ShuffleServer produces a side effect: the Shuffle’s KeyClass, ValueClass and KeyComparator need to be passed to ShuffleServer.
3.3 How does ShuffleServer combine?
Combine is generally a user-defined operation, so ShuffleServer is prohibited from performing combine operations. If combine is performed on the Reduce side, wouldn’t it violate our theme of avoiding spill to disk on the task side? In fact we don’t have to use ExternalAppendOnlyMap for combine. If the Records obtained from ShuffleServer are sorted by key, it means that the same keys have been organized together, and only a small amount of memory is needed to combine.
3.4 How does Writer write?
Just write it the way we have it.
3.5 How does Reader read?
Currently, Uniffle’s shuffle reader uses blockid as the read mark, which makes it easy to verify whether an accurate and complete records are obtained. For remote merge, MergeManager has merged the original Block collection into a new sequence sorted records by key. Therefore, the blockid generated by the map segment cannot be used:
We will use a new way to read Records. When MergerManager performs global Merge, an index will be generated. Reader will read according to this index.
Note: In principle, using key as a read index is more semantic, and the first version of the demo program was also implemented by this way. However, this proposal was not friendly enough to deal with the problem of data skew, so gave up the plan.
4 Scheme Design
4.1 Basic procedures for RemoteMerge

The following introduces the process of Remote Merge:
- (1) Register
When AM/Driver calls the registerShuffle method, it will additionally register keyClass, valueClass and keyComparator. This information is mainly used by ShuffleServer to parse and sort the Record during merging. - (2) sendShuffleData
The sendShuffleData logic is basically consistent with existing RSS tasks. The only difference is the use of unified serializers and deserializers, which ensures that ShuffleServer can parse the Record normally no matter which framework it is. - (3) buffer and flush
The shuffle server will store the data in the cache, or cache it to the local file system or remote file system through the flush manager. The logic of the original ShuffleServer is still reused here. - (4) reportUniqueBlocks
A new API is provided, reportUniqueBlocks. The Reduce end will deduplicate the blocks generated by the map, and then send the valid block set to ShuffleServer through reportUniqueBlocks. After ShuffleServer receives a valid blocks collection, it will trigger Remote Merge. The results of Remote Merge will be written to the bufferPool like a normal block, and may be flushed to disk when necessary. The result generated by RemoteMerge is an ordinary block, but for convenience of explanation, it is called merged block here. The merged block records the results sorted by key, so when reading the merged block, you need to read it in ascending order in the order of blockid. - (5) getSortedShuffleData
Reduce will read merged blocks in the order of block serial numbers, and then choose when to use them for reduce calculations based on certain conditions.
4.2 Analyze the process from the perspective of Record
We can use WordCount as an example to explain the flow of record in the entire process. In this example, there are two partitions and one reduce, that is, one reduce processes the data of two partitions.
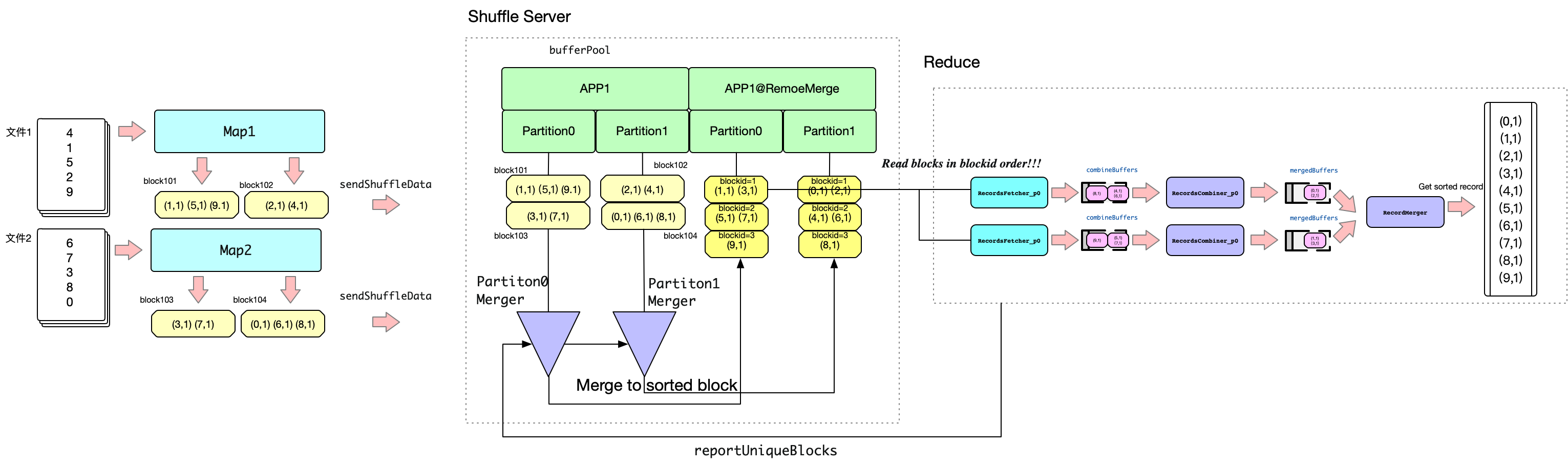
- (1) MAP side
After the document data is processed on the map side, it will be sorted. For Map1, since there are two partitions, records with odd numbers as keys will be written to block101, and records with even numbers as keys will be written to block102. The same goes for Map2. Note that the Records in the block here are all sorted. - (2) Map side sends data
The map side sends the block to ShuffleServer through sendShuffleData, and ShuffleServer stores it in the bufferPool.
What I mean here is that when registering, the app named APP1 will also be registered, and the app named APP1@RemoteMerge will also be registered, which will be introduced later. - (3) ShuffleServer side Merge
After reduce is started, reportUniqueBlocks will be called to report the available block set, and the corresponding partition in ShuffleServer will be triggered to merge. The result of Merge is a globally sorted record collection under this partition.
Then the question is where are the results of merge stored? The merge process occurs in memory. Whenever a certain number of records are merged, the results are written to a new block. In order to distinguish it from the original appid, this group of blocks will be managed in an appid ending with “@RemoteMerge”. The blockid of this new group of blocks increases from 1 and is sorted globally. That is, the records inside each block are sorted, and the records with blockid=1 must be less than or equal to the records with blockid=2. - (4) Reduce end reading
According to the previous analysis, the reduce side only needs to read the block managed by the appid ending with “@RemoteMerge”. When reduce reads a block, it starts from the block with blockid=1 and reads in the order of blockid. We know that when reduce performs calculations, it is calculated in order. Since the data we obtain on the ShuffleServer side is already sorted, we only need to obtain a small amount of data from the ShuffleServer side each time. This enables on-demand reading from the ShuffleServer side, which greatly reduces memory usage.
There are two special situations here, detailed in 5.5.
5 Plan
5.1 Unified serializer
Since Merge needs to be performed on the ShuffleServer side, a unified serializer that is independent of the computing framework needs to be extracted. Two types of serializers are extracted here: (1) Writable (2) Kryo. Writable serialization is used for classes that handle the org.apache.hadoop.io.Writable interface, used in the MR and TEZ frameworks. Kryo can serialize most classes and is generally used in the Spark framework.
5.2 RecordsFileWriter/RecordFileReader
Provides abstract methods for processing Records
5.3 Merger
Provides basic Merger service to merge multiple data streams according to key. Minimum heap K-way merge sorting is used to merge and sort the data streams that have been partially sorted.
5.4 MergeManager
Used to merge Records on the server side.
5.5 Tools for reading sorted data
Generally speaking, when the Reduce side reads data, it can be sent directly to downstream calculations. But there are two special situations:
(1) For situations where it is necessary to combine in Merge, we need to wait for all the same keys to arrive before combining, and then send them to the downstream.
(2) For spark and tez, the reduce end can read data from multiple partitions. Therefore, we need to merge the data of multiple partitions again on the reduce side, and then send it to the downstream.
RMRecordsReader is a tool for reading sorted data. The general structure is as follows:
The figure depicts a situation where a single Reduce processes two partitions. The RecordsFetcher thread will read the block of the corresponding partition and then parse it into Records. Then send it to combineBuffers. RecordCombiner reads Records from combineBuffer. When all records of a certain key are collected, a combine operation is performed. The result will be sent to mergedBuffer. RecordMerge will obtain all mergedBuffers and then merge and sort them again in memory. Finally, the global sorting results are obtained for downstream.
5.6 Framework adaptation
Compatible with MR, Tez, and Spark architectures.
I has used online application to conduct large-scale stress testing on MR and Tez. Spark has currently only tested some basic examples and still needs a lot of testing.
5.7 Isolated classloader
For different versions of keyclass, valueclass and comparatorclass, use isolated classloaders to load them.
Chinese version document: https://zhengchenyu.github.io/2023/12/25/RSS-%E8%BF%9C%E7%A8%8BMerge%E7%9A%84%E8%AE%BE%E8%AE%A1/
机器学习-3.2-非监督学习之主成分分析.md
主成分分析(PCA)
严格上说PCA应该算是一种降低维度的方法,这里暂时归类为非监督学习中。
1 PCA理论简述
PCA的主要思路是将\(n\)维数据降维到\(k\)维子空间中,以滤除不需要的噪声或没有意义的特征信息。譬如我们有汽车的一组运行数据,包括专项半径、速度等。其中就一个用英里/每小时和千米/每小时描述的速度,很明显两者是呈线性关系的,只是因为舍入带来一些误差,对于这样的问题我们完全可以将这个\(n\)维数据移到\(n-1\)维子空间中。
再举一个例子,对于RC直升机驾驶员的调查,\(x _1\)表示飞行员驾驶技能,\(x _2\)表示他对驾驶直升飞机的爱好程度。因为RC直升机很难驾驶,因此我们可以认为只有任务对驾驶RC直升机感兴趣的驾驶员才能学好它。因此,\(x _1\)和\(x _2\)有强相关性。如下图所示,我们可以假定一个方向\(u _1\),用来表示\(x _1\)和\(x _2\)两个属性,仅仅在其垂直轴\(u _2\)上有少量噪声。
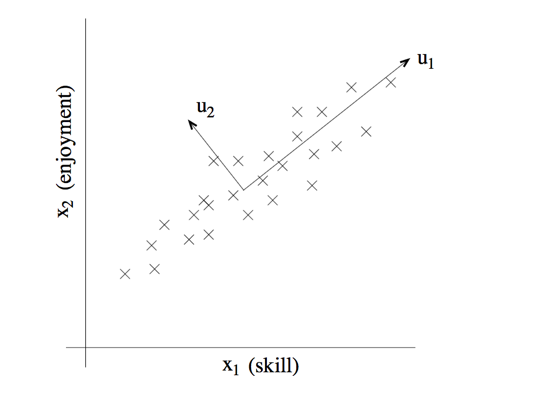
对数据降维之前,我们需要对数据进行初始化,主要是一个归整的过程,将均值归整为0,将方差归整为1。依次按照如下公式进行归整。
- \(\mu = \frac{1}{m}\sum\limits _{i = 1}^m {x^{(i)}} \)
- \({x^{(i)}}: = {x^{(i)}} - \mu \)
- \({\sigma ^2} = \frac{1}{m}\sum\limits _{i = 1}^m {(x^{(i)})^2} \)
- \({x^{(i)}}: = {x^{(i)}}/\sigma \)
归整后的数据如下,可以知道如果\(x\)在向量\(u\)的投影最大。说明\(u\)在对\(x _1\)和\(x _2\)的降维过程中带来的损失越小。
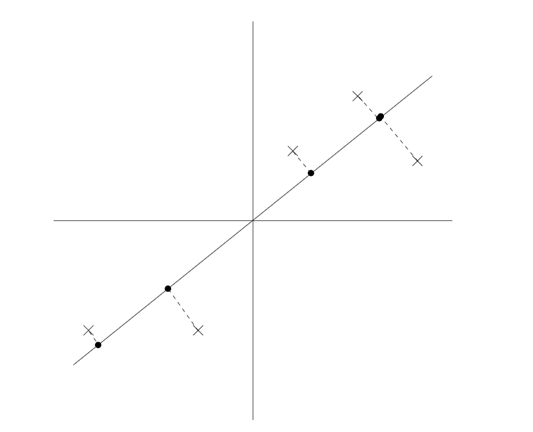
因此,可以需要保证下式最大:
$$\frac{1}{m}\sum\limits _{i = 1}^m {{{({x^{{{(i)}^T}}}u)}^2}} = \frac{1}{m}\sum\limits _{i = 1}^m {{u^T}{x^{(i)}}{x^{{{(i)}^T}}}u} = {u^T}(\frac{1}{m}\sum\limits _{i = 1}^m {{x^{(i)}}{x^{{{(i)}^T}}}} )u$$问题也就转变为找到一组\(u\)使得,上式最大的问题。然后我们假定\(u\)为一组标准正交基,因此\(||u||=1\)。因此问题可写为如下形式:
$$\begin{gathered} & \max {u^T}(\frac{1}{m}\sum\limits _{i = 1}^m {{x^{(i)}}{x^{{{(i)}^T}}}} )u \hfill \\\ s.t.: & ||u|| = 1 \hfill \\\ \end{gathered} $$组成其拉格朗日函数如下:
$$L(u,\lambda ) = {u^T}(\frac{1}{m}\sum\limits _{i = 1}^m {x^{(i)}x^{(i)^T}} )u - \lambda (||u|| - 1)$$这里我们设\(\Sigma=\frac{1}{m}\sum\limits _{i = 1}^m {x^{(i)}{x^{(i)^T}}}\),然后对\(u\)求导数,易得:
$${\nabla _u}L(u,\lambda ) = \sum u - \lambda u = 0$$因此,我们知道\(\lambda\)为\(\Sigma\)的特征值,\(u\)为对应的特征向量。恰好为我们要求的向量\(u\)。得到一组新的正交基后,我们就可以映射到新的空间中了。具体如下:
$${y^{(i)}} = {(u _1^T{x^{(i)}},u _2^T{x^{(i)}},...,u _k^T{x^{(i)}})^T}$$2. PCA的奇异值分解
由于一般为\(n*n\)的维度,因此往往是一个很大的矩阵。对于维度很大,样本数目要少于维度很多的数据,这样计算往往有不够划算。因此我们可以通过求解\(x\)的特征值的方法来求节\(\Sigma\)的特征向量。
由于求矩阵的单位特征向量,可以不考虑1/m这个系数。
假如对\(x\)进行奇异值分解,其中\(U\)和\(V\)均为酉阵,\(\Lambda\)为对角阵。另外值得注意的是酉阵的可逆矩阵与转置矩阵相同。具体分解形式如下:
$$x = U\Lambda {V^T}$$因此可以得到:
$$\Sigma = x{x^T} = U\Lambda {V^T}V\Lambda {U^T} = U{\Lambda ^2}{U^T}$$ $$\Sigma U = {\Lambda ^2}{U^T}$$因此可以知道\(\Sigma\)的特征向量对应着\(x\)的奇异值分解中的\(U\)。同时\(\Sigma\)的特征值为\(x\)的奇异值的平方。
3 源码实现
我们对k临近算法中的手写数字识别做分析。我们首先对数字映射到三维空间中,然后进行展示。然后在映射到样本数量维度的空间,实现对数字的识别。值得一提是,PCA可以将多种无法直接展示的多维数组转化为三维数据,然后更直观地进行分析。
1 | import numpy as np |
500个测试样本中,有27个识别错误,具体识别率为94.6%。这与未使用PCA降维的完全一致。该例子似乎尚未体现到PCA有什么优势,以后有机会在分析。当然如果映射到三维空间,识别率仅仅为75.2%,因此不要过度降维。下面是一个展示到部分数据的三维图。
考虑到颜色,图片只显示部分类别数据。
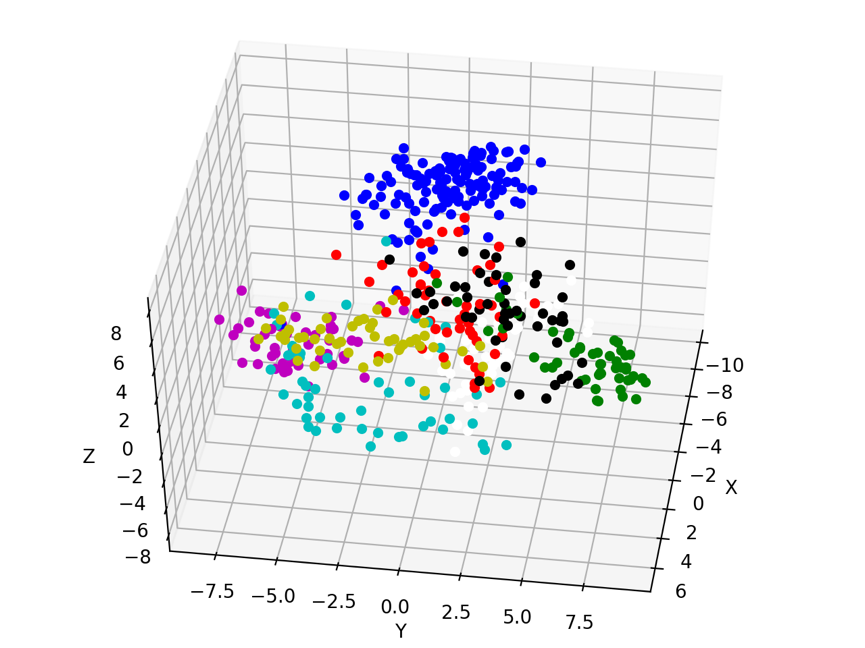
4 参考文献
- cs229-note10
- 机器学习实战
机器学习-2.8-监督学习之k近邻算法
K近邻算法
K近邻算法是一种常用的监督学习算法。其工作机制非常简单: 给定测试样本,基于某种距离度量找到与测试样本最近的k个训练样本,然后可以根据k个样本决定分类。譬如可以选择k个样本中最多的测试样本进行分类。
下面我们使用k近邻算法识别手写数字。手写数字为一个32*32维的向量,我们可以把它看成一个1024维的向量。然后任意两个样本的距离完全可以通过计算1024维上的两个点之间的距离获得,然后我们可以找到与测试样本距离最近的的10个样本,其中最多的分类我们认为他就是测试样本的分类。具体代码如下:
1 | import numpy as np import os from numpy import linalg global g_train global g_label def makeTrain(dir): dirs=os.listdir(dir) files = filter(lambda item:not os.path.isdir(item), dirs) mat = [] label = [] for file in files: arr = [] f = open(dir+"/"+file) while True: line = f.readline() if not line: break for i in range(len(line)-1): # line[len(line)]='\n' arr.append(int(line[i])) mat.append(arr) label.append(int(file.split("_")[0])) return (np.array(mat),np.array(label)) def testClassify(dir): dirs=os.listdir(dir) files = filter(lambda item:not os.path.isdir(item), dirs) mat = [] right=0 wrong=0 for file in files: arr = [] f = open(dir+"/"+file) while True: line = f.readline() if not line: break for i in range(len(line)-1): # line[len(line)]='\n' arr.append(int(line[i])) mat.append(arr) testLabel = file.split("_")[0] label=classify(np.array(arr),10) if testLabel == label: right=right+1 else: wrong=wrong+1 print("right=",right,", wrong=",wrong) def classify(vec,k): # 计算各个训练数据与测试数据的距离 m = len(g_label) dis = [] for i in range(m): dis.append([linalg.norm(vec-g_train[i]),g_label[i]]) dis = sorted(dis, key=lambda v:v[0]) # 计算相似度最高的k个值,这里写入map做累积 dic = {} for j in range(k): if not str(dis[j][1]) in dic: dic[str(dis[j][1])]=1 else: dic[str(dis[j][1])]=dic[str(dis[j][1])]+1 return max(dic.items(), key=lambda x: x[1])[0] if __name__ == "__main__": # 1 formate trainning date (g_train,g_label) =makeTrain("/Users/zcy/Desktop/study/git/mlearning/res/trainingDigits1") # 2 test testClassify("/Users/zcy/Desktop/study/git/mlearning/res/testDigits1") |
计算946个测试样本集,有926个为正确计算,20个为错误计算,识别为97.89%
机器学习-3.1-非监督学习之聚类.md
聚类问题
本节主要介绍非监督学习的聚类算法。
1 常见的聚类算法
聚类问题中,我们给定训练集合\(\{ {x^{(1)}},{x^{(2)}},…,{x^{(m)}}\}\),目的是将训练样本聚合几个类中。由于问题过程中,\(y\)并没有指定,所以这是一个非监督问题。
1.1 k-means
下面介绍k-means算法。算法的主要流程如下:
- (1) 随机初始化重心\(\{ {x^{(1)}},{x^{(2)}},…,{x^{(m)}}\}\)(假设有k个分类)
- (2) 根据当前的\(\{ {x^{(1)}},{x^{(2)}},…,{x^{(m)}}\}\),计算距离每个样本距离最近的中心,即为所属类。
- (3) 然后根据2中得到新的所属类关系,更新一组新的重心值。
- (4) 重复2,3直到某个截止条件。
下面我们随机制造以三个点为高斯分布的一组数据,试图从该组数据完成聚类操作,具体代码如下:
1 | import numpy as np |
下面是随机产生的基于(1,1),(2,2),(1,2)的高斯分布。
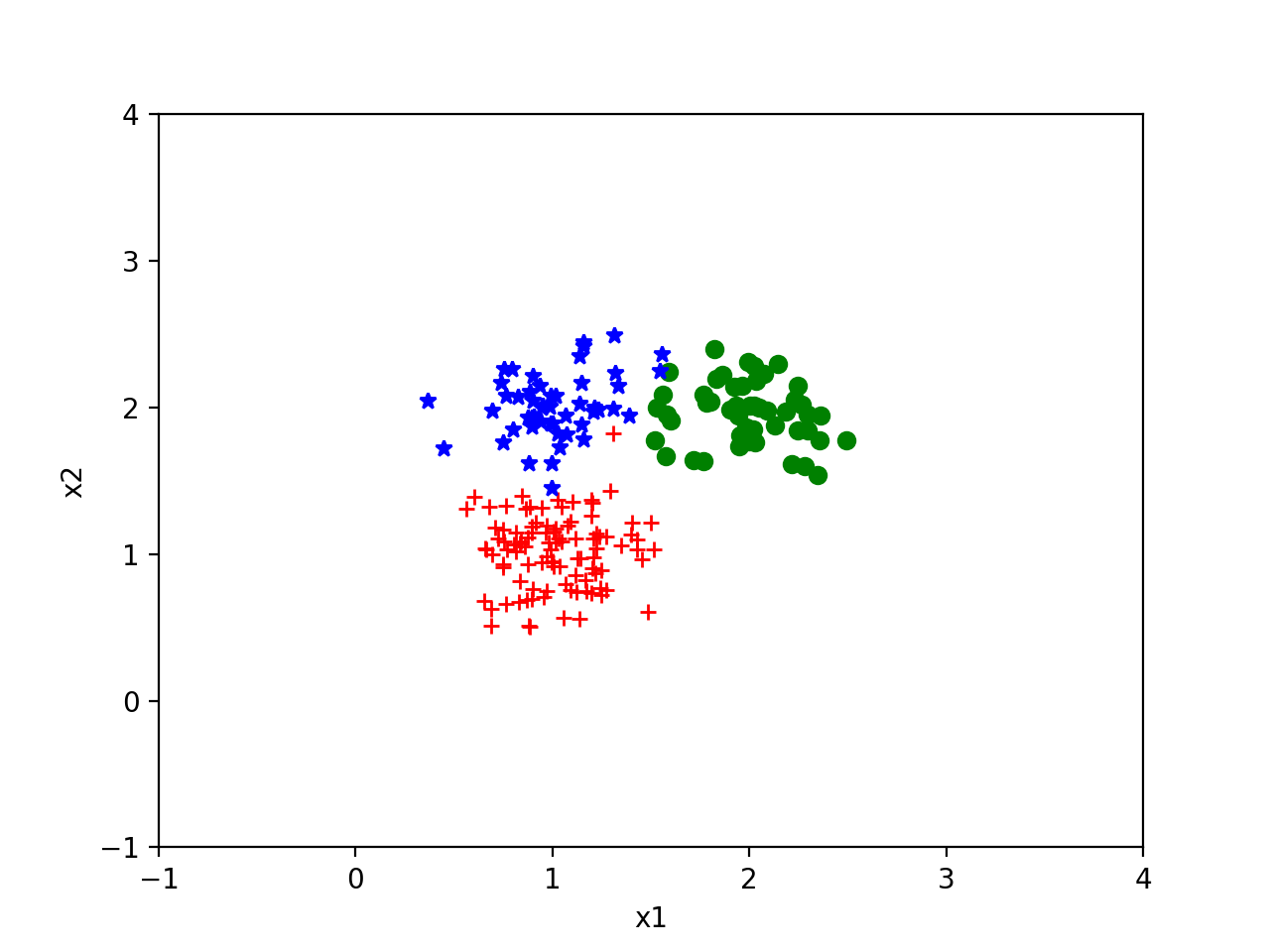
然后我们从(0.0,3.0),(3.0,3.0),(0.0,0.0)开始迭代得到如下效果,可以看出结果还是非常理想的。
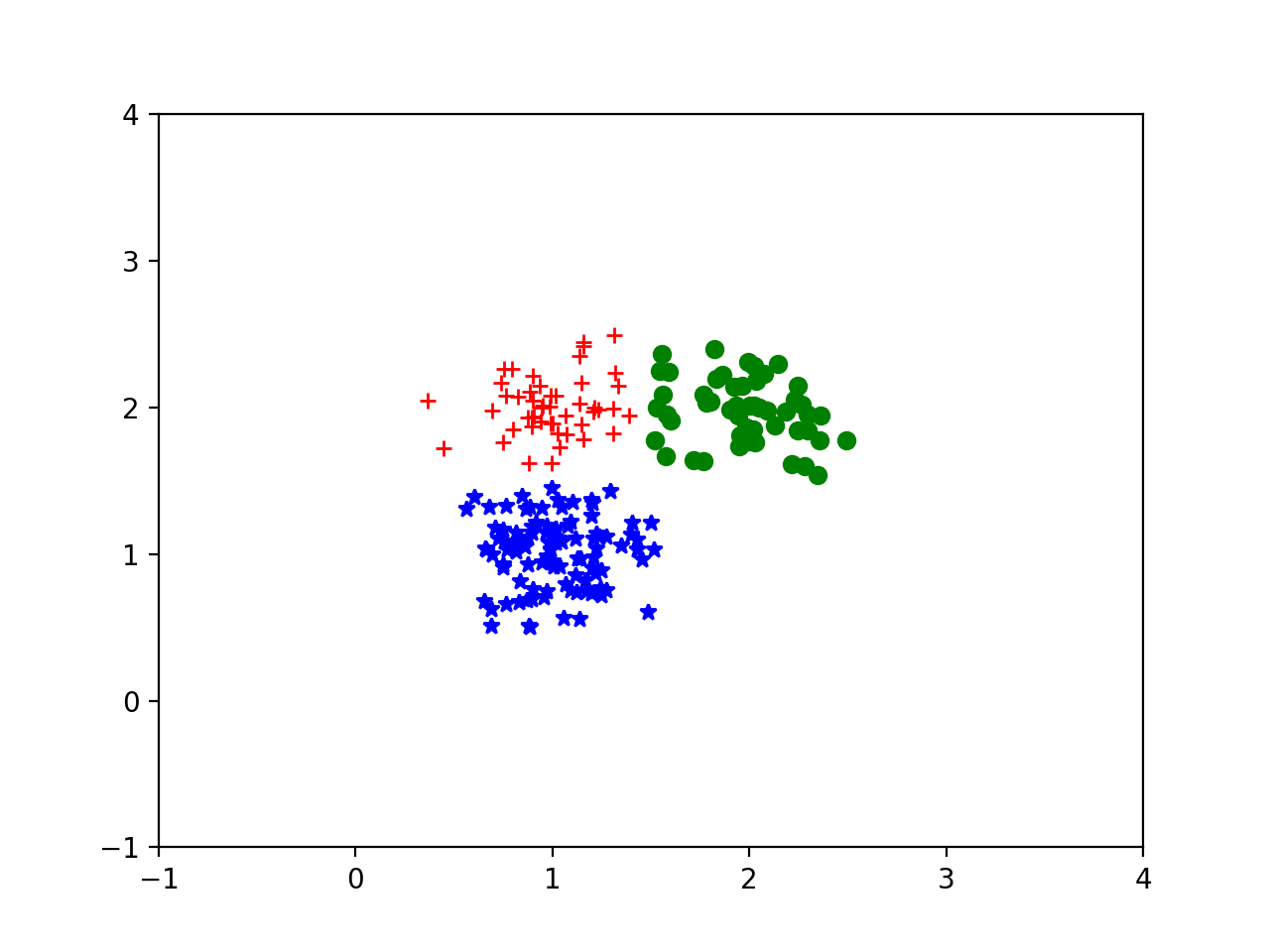
然后我们试图从(0.0,10.0),(2.0,3.0),(0.5,5.0)开始迭代,则会得到这样的结果。
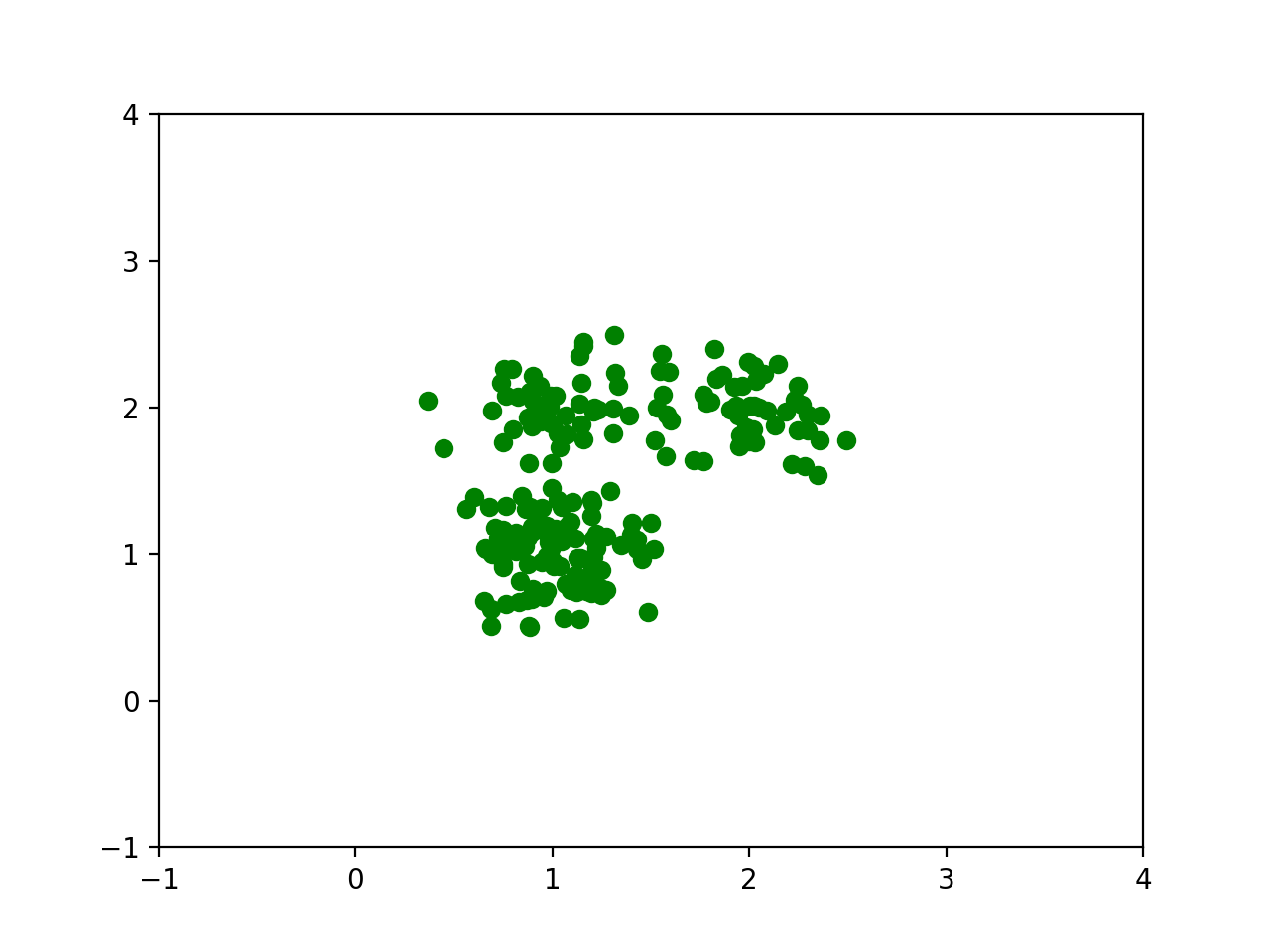
可以从上文中看出,k-means对初始值的敏感度很高。对于现实问题,也许我们并不知道训练样本中本身存在多个分类,我们可以设置多个分类,如果某一个分类里面的样本过少,就删除分类,这样就不再依赖于事先知道分类的数量了。
1.2 高斯混合聚类
假设我们的分类都服从于各自的高斯分布,我们试图从样本中对其分类。该问题与之前的高斯判别分析类似,区别仅仅在于该问题没有样本标签。
我们设置z表示样本的距离分类,可以知道他服从一个多项式分布, \({z^{(i)}} \sim Multinomial(\phi )\),其中。然后已经\(z\)之后,\(x\)服从的是一个高斯分布,\({x^{(i)}}|{z^{(i)}} = j \sim N({\mu _j},{\Sigma _j})\)。
下面直接写出算法过程(具体的算法推导见EM算法小节):
- (1) 随机初始化\(\phi\),\(\mu\),\(\Sigma\)。
- (2) 遍历样本,计算得\([w _j^{(i)}\),如下:
- (3) 根据计算得到的\(w\)重新更新各个分类的分布,如下:
- (4) 重复2,3知道达到截止条件。
下面我们制作一组由三个高斯分布组成的样本数据,对其进行聚类。代码如下:
1 | # coding=utf-8 |
我们分别以[6.0, 6.0], [4.0, -1.0], [-2.0, 2.0]为均值,以[[0.1,0],[0,0.1]],[[0.1,0],[0,0.1]],[[0.1,0],[0,0.1]]为标准差生成一组高斯分布如下:
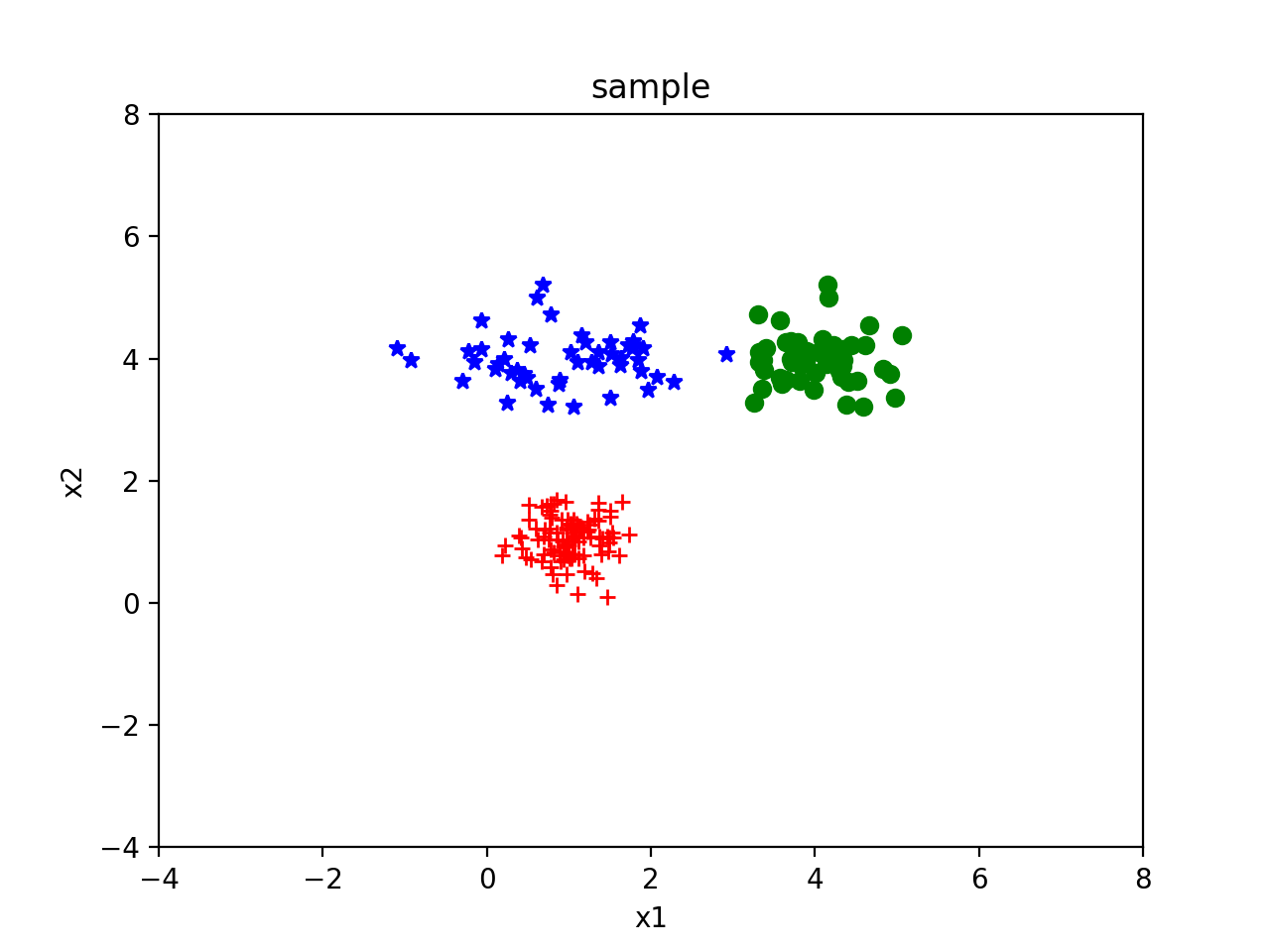
然后通过训练的到的训练均值分别为[4.00729834, 3.99848889], [1.01089497, 1.05225006], [0.92949217, 4.18380895]]。标准差为[[0.23196114,-0.01896477],[-0.01896477, 0.17165715]], [[0.10205901, 0.00157192], [0.00157192, 0.11477843]], [[0.7010517, 0.04783335], [0.04783335, 0.51147277]]。具体结果如下:
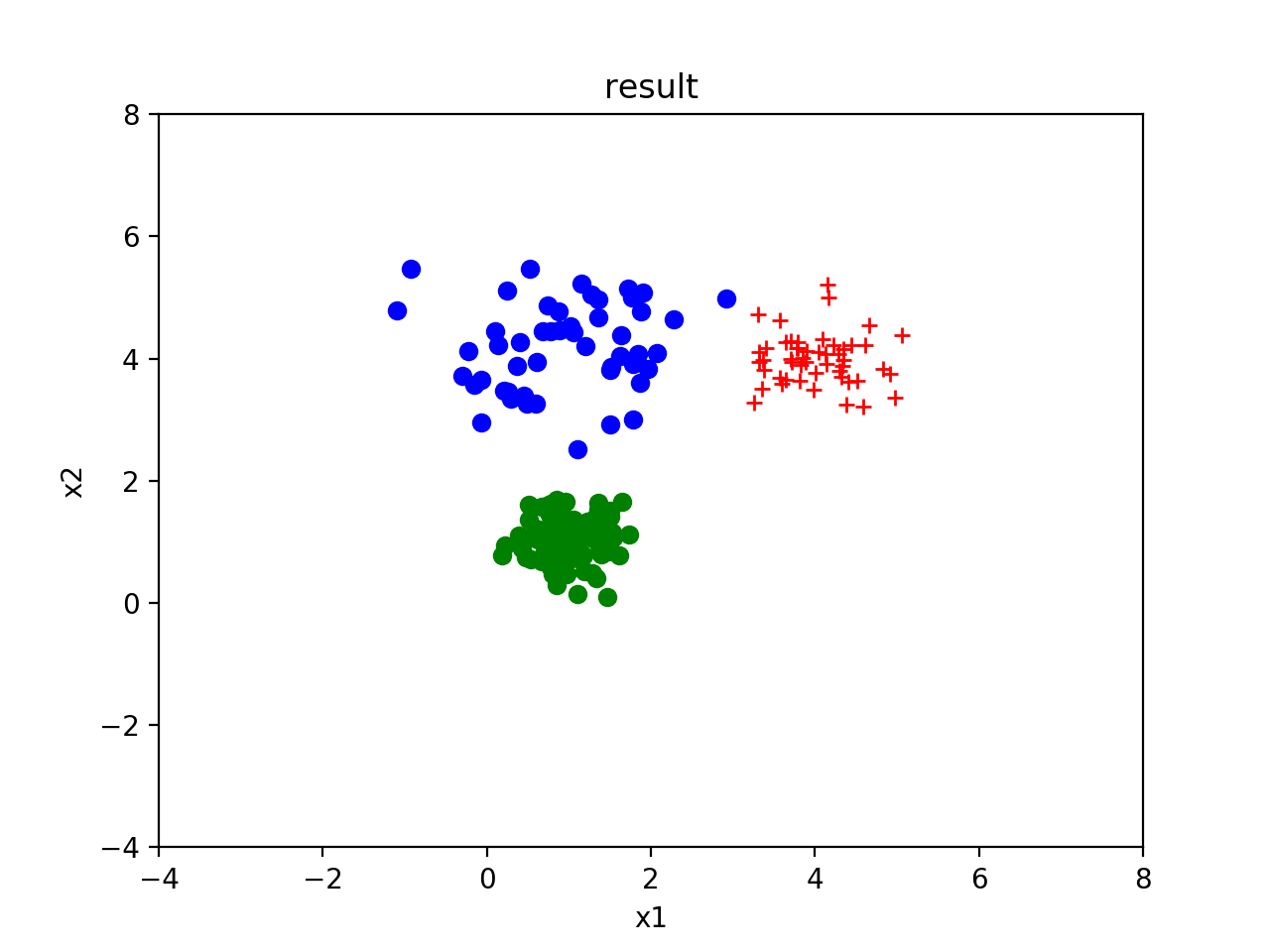
当且仅当x为常数的时候,上式等号成立。对于严格凹函数,则正好相反。
2.2 EM算法模型建立
假定我们的数据有\(k\)个分类。我们聚类的目标是,样本在自己分类中出现的概率最大。或者换句话说,让其在所属分类的分布(可以用高斯分类假想该问题)中出现的概率最大。可是对于非监督学习问题,我们不知道具体的分类。因此,我们可以将模型假定为找到给定参数\(\theta\)对应分布,是的\(x\)在分布中出现的概率足够大,这说明(\theta\)对应的分布能够充分的表示某一组分类。因此可以构造如下的最大释然函数:
$$\ell (\theta ) = \sum\limits _{i = 1}^m {\ln p({x^{(i)}};\theta )} $$进入推导最大释然函数:
$$\ell (\theta ) = \sum\limits _{i = 1}^m {\ln \sum\limits _{j = 1}^k {p({x^{(i)}},{z^{(j)}};\theta )} } = \sum\limits _{i = 1}^m {\ln \sum\limits _{j = 1}^k {{Q _i}({z^{(i)}} = j)\frac{{p({x^{(i)}},{z^{(i)}} = j;\theta )}}{{{Q _i}({z^{(i)}} = j)}}} } $$上面\(k\)为分类的个数。对上面的\({Q _i}\)为一个概率分布,有:
$$\sum\limits _{j=1} ^k {{Q _i}({z^{(i)}}=j)}=1$$对于\(f(x) = \ln x\),我们知道\(f’’(x) = - \frac{1}{x^2}\),可知其为一个严格凹函数。上式可以写成:
$$\ell (\theta ) = \sum\limits _{i = 1}^m {f(E[\frac{{p({x^{(i)}},{z^{(i)}} = j;\theta )}}{{{Q _i}({z^{(i)}} = j)}}])}$$根据Jensen不等式,我们有:
$$\ell (\theta ) = \sum\limits _{i = 1}^m {f(E[\frac{p({x^{(i)},{z^{(i)}};\theta )}}{{Q _i}({z^{(i)}} = j)}])} \geqslant \sum\limits _{i = 1}^m {E[f(\frac{p({x^{(i)}},{z^{(i)}} = j;\theta )}{{Q _i}({z^{(i)}} = j)})]} = \sum\limits _{i = 1}^m {\sum\limits _{j = 1}^k {{Q _i}({z^{(i)}} = j)\ln (\frac{p({x^{(i)}},{z^{(i)}} = j;\theta )}{{Q _i}({z^{(i)}} = j)})} }$$因此我们得到最大释然函数的下确定,即为上式子中后面的部分。我们只要保证下确定随着迭代的方向单调递增即可,具体的要保证\(\ell ({\theta ^{(t + 1)}}) \geqslant \ell ({\theta ^{(t)}})\)。这里我们设\(low(\theta )\)是已知\({Q _i}\)的情况下,最大释然函数的下确定关于\(\theta\)的函数。我们看如下公式:
$$\ell ({\theta ^{(t + 1)}}) \geqslant low({\theta ^{(t + 1)}}) \geqslant low({\theta ^{(t)}}) = \ell ({\theta ^{(t)}})$$事实上,我们只要保证上面的式子我们就可以保证迭代方向是正确的。其中,第一个不等号是必然成立的。那我们分头来构造条件是后面的式子成立。
首先如果保证最后一个等号的成立,根据Jensen不等式,只有自变量为常数才能事等号成立,这样我们设置如下式子(其中\(c\)为常数):
然后对上面的式子按照分类累积求和得:
$$\sum\limits _{j = 1}^k {p({x^{(i)}},{z^{(i)}} = j;\theta )} = c\sum\limits _{j = 1}^k {{Q _i}({z^{(i)}} = j)} = c$$因此得:
$${Q _i}({z^{(i)}} = j) = \frac{{p({x^{(i)}},{z^{(i)}} = j;\theta )}}{{\sum\limits _{j = 1}^k {p({x^{(i)}},{z^{(i)}} = j;\theta )} }} = p({z^{(i)}}|{x^{(i)}};\theta)$$这样我们完成EM算法的E步骤。然后解决中间大于等于号的问题。这个就比较好解决了,我们只需要计算下确定函数关于\(\theta\)求最大值,最大值对应的参数,可保证不等号的成立,即为迭代的正确方向。具体公式如下:
$$\theta = \arg {\max _\theta }\sum\limits _{i = 1}^m {\sum\limits _{j = 1}^k {{Q _i}({z^{(i)}} = j)\ln \frac{{p({x^{(i)}},{z^{(i)}} = j;\theta )}}{{{Q _i}({z^{(i)}} = j)}}} }$$综上,我们来重新整理一下EM算法,具体如下:
- (1) 初始化相关参数
- (2) E步骤:计算\({Q _i}\),如下:
- (3) M步骤:更新参数\(\theta\),如下:
(4) 重复2,3直到截止条件
注: 上面的例子是不断的更新迭代\({Q _i}\)和\(\theta\)。我们完全可以使用梯度上升发不断从各个方向更新\({Q _i}\)和\(\theta\),来完成最大值的逼近。
2.3 混合高斯分布的公式推导
对比之前的内容,我们可以发现混合高斯分布的聚类问题为EM算法的一个特例。可以通过通用的EM算法来证明,下面我们来证明这一过程。
注: 这里只简单地提示计算,不展开了,因为与之前的高斯判别分析类似。
根据上一节,我们已得到E步骤的公式:
然后对于M步骤,我们将\({Q _i}\)为一个已知值的方式对\(\theta\)进行求导,从而求得下确定的最大值,记为下一个迭代值。将最大释然函数展开记为如下函数:
$$\ell (\theta ) = \sum\limits _{i = 1}^m {\sum\limits _{j = 1}^k {{Q _i}({z^{(i)}} = j)\ln (\frac{{p({x^{(i)}},{z^{(i)}} = j;\theta )}}{{{Q _i}({z^{(i)}} = j)}})} } = \sum\limits _{i = 1}^m {\sum\limits _{j = 1}^k {w _j^{(i)}\ln (\frac{{\frac{1}{{{{(2\pi )}^{\frac{n}{2}}}|\Sigma {|^{\frac{1}{2}}}}}\exp ( - \frac{1}{2}{{({x^{(i)}} - {\mu _j})}^T}{\Sigma ^{ - 1}}({x^{(i)}} - {\mu _j})){\phi _j}}}{{w _j^{(i)}}})} } $$然后对\(\phi\),\(\mu\),\(\Sigma\), 即可得到M步骤的更新公式。
机器学习-2.7-监督学习之支持向量机
支持向量机(SVM)
1 Margins(间隔)
对于一个逻辑回归问题,\(p(y = 1|x, \theta )\)可以通过下面的式子表示:
$${h _ \theta }(x) = g({\theta ^T}x)$$\(\theta^Tx\)越大, \(g({\theta ^T}x)\)就越大, 我们就有可高的信心认为\(y=1\)。 同理假如\({ h _\theta }(x) << 0\), 我们就有很强的信息认为\(y=0\)。 (注: >> 表示远大于,<<表示远小于)
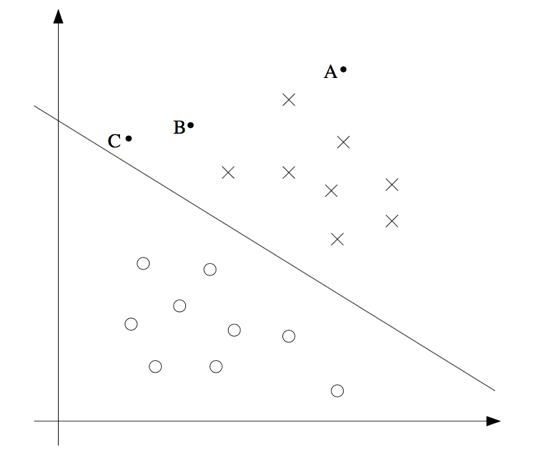
上面这张图,x为正样本,o为负样本。中间的直线是分割超平面。上面图中,我们可以知道对于样本A,我们有很强的信心认为\(y=1\)。然后对于C却接近边界,如果稍微改变分割超平面,就可能导致\(y=0\)。因此,越远离分割超平面的样本,我们越有信息获得他的分类。
2 函数化与几何间隔
区别与之前的逻辑回归。引入支持向量机,我们需要重新定义符号。对于SVM问题, \(y \in \{ - 1,1\} \),定义函数为:
$${h_{w,b}}(x) = g({w^T}x + b)$$对于\(z = {w^T}x + b > 0\), 有\({h _ \theta }(x) = 1\)。 否则\({h _ \theta }(x) = -1\)。
函数化几何间隔,有如下公式:
$${\hat \gamma ^{(i)}} = {y^{(i)}}({w^T}x + b)$$上式很容易理解,对于正样本\(y=1\),对于负样本\(y=-1\)。因此可以得到\({\hat \gamma ^{(i)}}\)为一个正值。我们可以就可以定义其为几何间隔,可以理解为距离。根据上一节的分析,一个超平面对样本分割的好坏,取决于距离超平面最近的样本。因为我们定义最小间隔如下:
$$\hat \gamma = {\min _{i = 1,...,m}}{\hat \gamma ^{(i)}}$$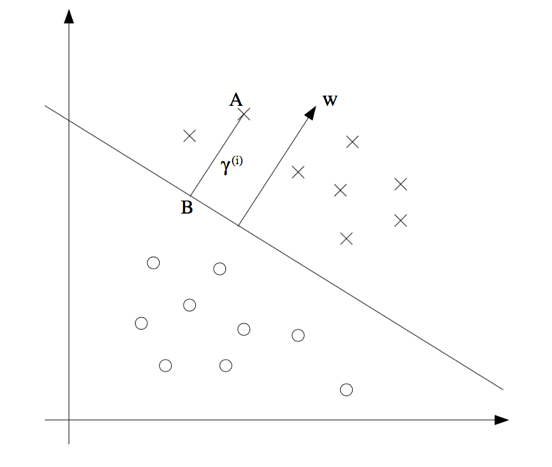
根据上图,我们知道向量\(w\)与超平面\({w^T}x + b = 0\)垂直。
在超平面找任意两点做直线,易证其与法向量內积为零。因此可知法向量与平面垂直。
我们可以得知B点的坐标为\({x^{(i)}} - {r^{(i)}}\frac{w}{||w||}\),我们将坐标代入超平面方程,有:
$${w^T}({x^{(i)}} - {r^{(i)}}\frac{w}{||w||}) + b = 0$$由于知道\(||w|| = {w^T}w\),有:
$${r^{(i)}} = {(\frac{w}{||w||})^T}{x^{(i)}} + \frac{b}{||w||}$$上面公式对应于正样本,对于负样本则需要加一个符号。因此我们可以综合得到如下公式:
$${r^{(i)}} = {y^{(i)}}({(\frac{w}{||w||})^T}{x^{(i)}} + \frac{b}{||w||})$$对于公式\({\hat \gamma ^{(i)}} = {y^{(i)}}({w^T}x + b)\)中,\(||w|| = 1\)的时候为几何间隔。上面的式子实际上就是定义了几何间隔。我们通过定义了向量\(w\)的尺度,这样不会因为选择w比例的不同而产生不同间隔的问题。
3 最优间隔分类器
以下假定我们样本数据是严格线性可分的,否则通过间隔最大化来计算就毫无意义了。
根据前面的分析,我们只需要找到超平面,使得间隔\(\hat \gamma \)最大即可。因此我们可以得到如下最优化问题:
$$\begin{gathered} & {\max _{w,b}}\gamma \hfill \\\ s.t.: & {y^{(i)}}({w^T}{x^{(i)}} + b) \geqslant \gamma , & i = 1,...,m \hfill \\\ & ||w|| = 1 \hfill \\\ \end{gathered} $$由于\(||w|| - 1 = 0\)不是凸函数,所以需要转化问题。
注> 为什么凸函数如此重要?因为对于一个凸优化问题,认为局部极小值点必为全局极小值点。对\(f(\lambda x + (1 - \lambda )y) \leqslant \lambda f(x) + (1 - \lambda )f(y)\),其中\(0 \leqslant \lambda \leqslant 1\),则\(f(x)\)为凸函数。可以理解为类似于\(y = {x^2}\)的图形。然而对于\(||w|| - 1 = 0\),具体为\(\sqrt {w _1^2 + w _2^2 + … + w _n^2} = 1\),二维的情景可以理解为一个圆,三维的话则为一个球。几何图形中,可以发现对于球或圆的上半部分正好与凸函数相反,因此不是凸函数。可以代入公式证明。
进一步转化问题,如下:
我们知道\(\gamma \)大小,取决于\(w\)和\(b\)的尺度,但是\(w\)和\(b\)的尺度的改变不会影响分配效果。因此我们固定\(\gamma \)为1。将问题转化为:
$$\begin{gathered} & {\min _{w,b}}\frac{1}{2}||w|{|^2} \hfill \\ s.t.: & {y^{(i)}}({w^T}{x^{(i)}} + b) \geqslant 1, & i = 1,...,m \hfill \\ \end{gathered}$$事实上对于这个问题,我们可以换一种几何意义的解释。如下图所示,我们需要找到\({w^T}x + b = 1\)和\({w^T}x + b = -1\)能够有效分割样本,并且保证是两个超平面之间间隔最大,即使\(\frac{2}{||w||}\)最大,也就意味着使\(\frac{1}{2}||w|{|^2}\)最小。同样我们可以得到上面的优化问题。
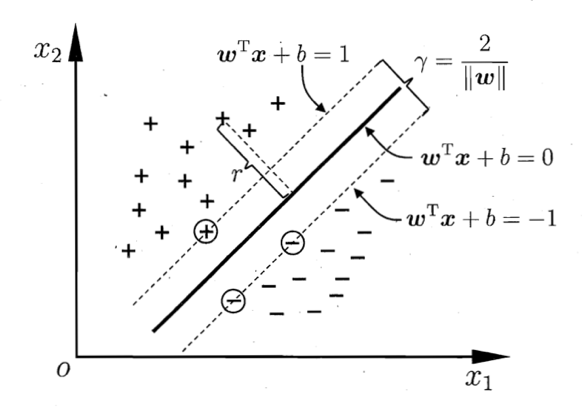
另外,我们距离超平面最近的点,即\(\gamma = 1\)的点,我们称之为支持向量。
4 拉格朗日对偶问题
在对计算上一级得到的优化问题之前,我们介绍一下拉格朗日对偶问题与KKT条件,以便于更容易解决问题。考虑一个通用的优化问题,如下:
$$\begin{gathered} & {\min _x}f(x) \hfill \\\ s.t.: & {g _i}(x) \leqslant 0, & i = 1,...,k \hfill \\\ & {h _j}(x) = 0, & j = 1,...,l \hfill \\\ \end{gathered} $$然后得到拉格朗日函数,如下:
$$L(x,\alpha ,\beta ) = f(x) + \sum\limits _{i = 1}^k {{\alpha _i}{g _i}(x)} + \sum\limits _{j = 1}^l {{\beta _i}{h _i}(x)} $$ $${\alpha _i} \geqslant 0$$对于我们的原始问题如何用拉格朗日函数表达呢?我们知道上面的拉格朗日后面两项的最大值为零。因此我们就可以将原始问题转化为以\({\alpha _i}\)和\({\beta _i}\)为参数情况下,求拉格朗日函数的最大值。具体转化为如下形式:
$${\theta _P}(x) = {\max _{\alpha ,\beta ;{\alpha _i} \geqslant 0}}L(x,\alpha ,\beta )$$然后我们上面的式子,关于x取极小值,就与目标问题一致了。原始为的最优解最终可以通过如下方式表述:
$${p^*} = {\min _x}{\theta _P}(x) = {\min _x}{\max _{\alpha ,\beta ;{\alpha _i} \geqslant 0}}L(x,\alpha ,\beta )$$我们这里可以写出其对偶问题:
$${\theta _D}(\alpha ,\beta ) = {\min _x}L(x,\alpha ,\beta )$$对偶问题的最优解如下:
$${d^*} = {\max _{\alpha ,\beta ;{\alpha _i} \geqslant 0}}{\theta _D}(\alpha ,\beta ) = {\max _{\alpha ,\beta ;{\alpha _i} \geqslant 0}}{\min _x}L(x,\alpha ,\beta )$$我们知道maxmin<minmax,所以我们得到如下式子:
$${d^*} = {\max _{\alpha ,\beta ;{\alpha _i} \geqslant 0}}{\min _x}L(x,\alpha ,\beta ) \leqslant {\min _x}{\max _{\alpha ,\beta ;{\alpha _i} \geqslant 0}}L(x,\alpha ,\beta ) = {p^\*}$$对于上面的式子只要\(f _i\)和\(g _i\)为凸函数,\(h _i\)为仿射函数,即可使等式两端相等。
$$\frac{\partial }{{\partial {x _i}}}L({x^*},{\alpha^*},{\beta^*}) = 0$$ $$\frac{\partial }{{\partial \beta }}L({x^*},{\alpha ^*},{\beta ^*}) = 0$$ $${\alpha ^*}{g _i}(x) = 0$$ $${g _i}(x) \leqslant 0$$ $${\alpha ^*} \geqslant 0$$仿射函数为线性函数加截距。
另外对于该问题的最优值必满足KKT条件。另外,上述拉格朗日函数对相应参数需要取得极值。最终得到如下条件:
KKT条件的原理暂时不深入,目前处于应用阶段,有时间再考虑具体原理。
5 最优边界分类器
将我们的优化问题转化为如下标准型:
$$\begin{gathered} & {\min _{w,b}}\frac{1}{2}||w|{|^2} \hfill \\\ s.t.: & - {y^{(i)}}({w^T}{x^{(i)}} + b) + 1 \leqslant 0, & i = 1,...,n \hfill \\\ \end{gathered} $$根据前面的说明,我们可以通过解对偶问题最优解来获得该问题的最优解。
首先写出拉格朗日对偶函数:
参数上一节的公式,这里\(w\)和\(b\)对应于\(x\)。我们需要关于\(w\),\(b\)求极小值,然后求得的极值点代入拉格朗日函数,然后求转化后的拉格朗日函数的极大值即可。
$$\frac{\partial }{{\partial w}}L(w,b,\alpha ) = w - \sum\limits _{i = 1}^m {{\alpha _i}{y^{(i)}}{x^{(i)}}} = 0$$ $$\frac{\partial }{{\partial b}}L(w,b,\alpha ) = \sum\limits _{i = 1}^m {{\alpha _i}{y^{(i)}}} = 0$$得到\(w = \sum\limits _{i = 1}^m {\alpha _i}{y^{(i)}{x^{(i)}}} \),\(\sum\limits _{i = 1}^m {\alpha _i}{y^{(i)}} = 0\)。然后将其代入拉格朗日函数:
$$L(\alpha ) = \frac{1}{2}{w^T}w - \sum\limits _{i = 1}^m {{\alpha _i}({y^{(i)}}({w^T}{x^{(i)}} + b) - 1)} $$ $$L(\alpha ) = - \frac{1}{2}{(\sum\limits _{i = 1}^m {{\alpha _i}{y^{(i)}}{x^{(i)}}} )^T}(\sum\limits _{i = 1}^m {{\alpha _i}{y^{(i)}}{x^{(i)}}} ) + \sum\limits _{i = 1}^m {{\alpha _i}} $$由于\({\alpha _i}\)和\({y^{(i)}}\)为变量,实际上面的式子就是一个\((Ax + By + Cz)(Ax + By + Cz)\)的问题。因此可以归纳为如下公式:
$$L(\alpha ) = - \frac{1}{2}\sum\limits _{i = 1}^m {\sum\limits _{j = 1}^m {{\alpha _i}{\alpha _j}{y^{(i)}}{y^{(j)}} < {x^{(i)}},{x^{(j)}} > } } + \sum\limits _{i = 1}^m {{\alpha _i}} $$问题就转化为:
$$\begin{gathered} & {\max _\alpha }L(\alpha ) = - \frac{1}{2}\sum\limits _{i = 1}^m {\sum\limits _{j = 1}^m {{\alpha _i}{\alpha _j}{y^{(i)}}{y^{(j)}} < {x^{(i)}},{x^{(j)}} > } } + \sum\limits _{i = 1}^m {{\alpha _i}} \hfill \\\ s.t.: & {\alpha _i} \geqslant 0 \hfill \\\ & \sum\limits _{i = 1}^n {{\alpha _i}{y^{(i)}}} = 0 \hfill \\\ \end{gathered} $$由于我们知道支持向量的间隔必须为1,因此我们可以根据其计算\(b\)。设支持向量的集合为S,对属于结合S的样本有\({y^{(i)}}({w^T}{x^{(i)}} + b) = 1\)。由于\(w = \sum\limits _{i = 1}^m {\alpha _i}{y^{(i)}}{x^{(i)}} \),又由于对所有的非支持向量,有\({\alpha _i} = 0\)。因此我们可以综合均值得到:
$$b = \frac{1}{{|S|}}\sum\limits _{i = 1}^S {({y^{(i)}} - \sum\limits _{j = 1}^S {{\alpha _j}{y^{(j)}} < {x^{(i)}},{x^{(j)}} > } )} $$6 正则化
关于之前的问题我们假定样本严格可分。但是实际上需要容忍一些误差。因此我们将公式修正为如下形式(C为常数):
$$\begin{gathered} & {\min _{w,b,\xi }}\frac{1}{2}||w|{|^2} + C\sum\limits _{i = 1}^m {{\xi _i}} \hfill \\\ s.t.: & {y^{(i)}}({w^T}{x^{(i)}} + b) \geqslant 1 - {\xi _i} & & i = 1,...,m \hfill \\\ & {\xi _i} \geqslant 0 & & & & i = 1,...,m \hfill \\\ \end{gathered} $$我们允许\({y^{(i)}}({w^T}{x^{(i)}} + b)\)小于1,但是不希望小太多。所以,我们需要保证\({\xi _i}\)的总和尽可能小,因此得上面的式子。然后我们可以得到拉格朗日公式如下:
$$L(w,b,\xi ,\alpha ,r) = \frac{1}{2}||w|{|^2} + C\sum\limits _{i = 1}^m {{\xi _i}} - \sum\limits _{i = 1}^m {{\alpha _i}({y^{(i)}}({w^T}{x^{(i)}} + b) - 1 + {\xi _i})} - \sum\limits _{i = 1}^m {{r _i}{\xi _i}} $$对\(w\),\(b\),\(\xi \), 求导得:
$$\frac{\partial }{{\partial w}}L(w,b,\xi ,\alpha ,r) = w - \sum\limits _{i = 1}^m {{\alpha _i}{y^{(i)}}{x^{(i)}}} = 0$$ $$\frac{\partial }{{\partial b}}L(w,b,\xi ,\alpha ,r) = - \sum\limits _{i = 1}^m {{\alpha _i}{y^{(i)}}} = 0$$ $$\frac{\partial }{{\partial {\xi _i}}}L(w,b,\xi ,\alpha ,r) = C - {r _i} - {\alpha _i} = 0$$可以得到\(w = \sum\limits _{i = 1}^m {\alpha _i}{y^{(i)}}{x^{(i)}}\),\(\sum\limits _{i = 1}^m {\alpha _i}{y^{(i)}} = 0\),\(C = {r _i} + {\alpha _i}\)。其中我们知道\({r _i} \geqslant 0\),所以也可得到\(0 \leqslant {\alpha _i} \leqslant C\)。带入拉格朗日函数得:
$$L(\alpha ) = - \frac{1}{2}\sum\limits _{i = 1}^m {\sum\limits _{j = 1}^m {{\alpha _i}{\alpha _j}{y^{(i)}}{y^{(j)}} < {x^{(i)}},{x^{(j)}} > } } + \sum\limits _{i = 1}^m {({r _i} + {\alpha _i}){\xi _i}} - \sum\limits _{i = 1}^m {{\alpha _i}({\xi _i}} - 1) - \sum\limits _{i = 1}^m {{r _i}{\xi _i}} $$我们对上面的式子乘以-1,转化为求最小值的问题,可以得到最终的优化问题:
$$\begin{gathered} & \min L(\alpha ) = \min \frac{1}{2}\sum\limits _{i = 1}^m {\sum\limits _{j = 1}^m {{\alpha _i}{\alpha _j}{y^{(i)}}{y^{(j)}} < {x^{(i)}},{x^{(j)}} > } } - \sum\limits _{i = 1}^m {{\alpha _i}} \hfill \\\ s.t.: & \sum\limits _{i = 1}^m {{\alpha _i}{y^{(i)}}} = 0 \hfill \\\ & 0 \leqslant {\alpha _i} \leqslant C \hfill \\\ \end{gathered} $$可以得到如下的KKT条件:
$${\alpha _i},{r _i} \geqslant 0$$ $${y^{(i)}}({w^T}{x^{(i)}} + b) - 1 + {\xi _i} \geqslant 0$$ $${\alpha _i}({y^{(i)}}({w^T}{x^{(i)}} + b) - 1 + {\xi _i}) = 0$$ $${\xi _i} \geqslant 0,{r _i}{\xi _i} = 0$$对于上述KKT条件我们可以转换为如下形式:
$${y^{(i)}}({w^T}{x^{(i)}} + b) \geqslant 1 , {\alpha _i} = 0$$ $${y^{(i)}}({w^T}{x^{(i)}} + b) \leqslant 1 , {\alpha _i} = C$$ $${y^{(i)}}({w^T}{x^{(i)}} + b) = 1 , 0 \leqslant {\alpha _i} \leqslant C$$很容易转化上面的式子,三个条件分别表示在比支持向量距离分割超平面远的样本,比支持向量距离分割超平面近的可容忍的误差样本,支持向量对应的样本。
7 SMO算法理论
这一节使用SMO算法解决上一节归纳出来的优化问题。
SMO算法的思想来自于坐标上升算法,坐标上升算法的主要思想是一次遍历一个变量,然后把其他变量当做是常亮,进在一个维度上优化。
然后对于我们之前的问题,有\(\sum\limits _{i = 1}^m {\alpha _i}{y^{(i)}} = 0\)。设置我们设\(\zeta = {\alpha _1}{y^{(1)}} + {\alpha _2}{y^{(2)}} = \sum\limits _{i = 3}^m {\alpha _i}{y^{(i)}} \),将\({\alpha _1}\)用\({\alpha _2}\)表达,然后得到关于\({\alpha _2}\)的二次函数,这样很容易取得极值。当所有样本满足KKT条件,且无法继续增加,我们就可以认为此刻取得最优值。
由于我们知道\(0 \leqslant {\alpha _i} \leqslant C\),所以我们可以求的其取值范围,我们可以将二维变量表述为一个方格内,具体如下:
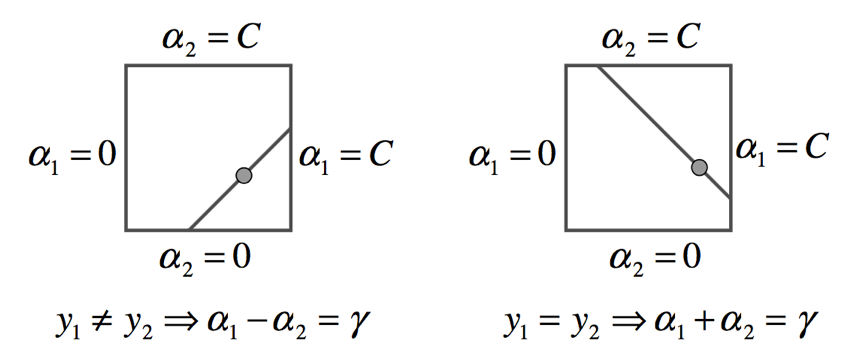
最多四种情况代入,经过求截距等一系列操作,可以将的取值范围归纳为下面的公式,其中L表示上界,H表示上界。
同号时有:
异号的时候有:
$$L = \max (0,\alpha _2^{old} - \alpha _2^{old})$$ $$H = \min (C,C + \alpha _2^{old} - \alpha _1^{old})$$进一步求解二次规划问题:
$$\psi ({\alpha _1},{\alpha _2}) = \frac{1}{2}\alpha _1^2k(1,1) + \frac{1}{2}\alpha _2^2k(2,2) + {y^{(1)}}{y^{(2)}}{\alpha _1}{\alpha _2}k(1,2) - {\alpha _1} - {\alpha _2} + {y^{(1)}}{\alpha _1}{v _1} + {y^{(2)}}{\alpha _2}{v _2} + M$$上式中\(k(i,j) = < {x _i},{x _j} > \)具体是核函数的简写,下节会介绍。\(M\)为与\(\alpha _1\),\(\alpha _2\)无关的参数。另外,\({v _1} = \sum\limits _{i = 3}^m {\alpha _i}{y^{(i)}}k(1,i) \),\({v _2} = \sum\limits _{i = 3}^m {\alpha _i}{y^{(i)}}k(2,i) \)。
我们设\(\zeta = {\alpha _1}{y^{(1)}} + {\alpha _2}{y^{(2)}}\),所以有\({\alpha _1} = {y^{(1)}}(\zeta - {\alpha _2}{y^{(2)}})\),代入上式:
$$\psi ({\alpha _2}) = \frac{1}{2}{(\zeta - {\alpha _2}{y^{(2)}})^2}k(1,1) + \frac{1}{2}\alpha _2^2k(2,2) + {y^{(2)}}(\zeta - {\alpha _2}{y^{(2)}}){\alpha _2}k(1,2) - (\zeta - {\alpha _2}{y^{(2)}}){y^{(1)}} - {\alpha _2} + (\zeta - {\alpha _2}{y^{(2)}}){v _1} + {y^{(2)}}{\alpha _2}{v _2} + M$$然后求导数:
$$\frac{\partial }{{\partial {\alpha _2}}}\psi ({\alpha _2}) = ({\alpha _2}{y^{(2)}} - \zeta ){y^{(2)}}k(1,1) + {\alpha _2}k(2,2) + {y^{(2)}}\zeta k(1,2) - 2{\alpha _2}k(1,2) + {y^{(1)}}{y^{(2)}} - {y^{(1)}}{y^{(2)}} - {y^{(2)}}{v _1} + {y^{(2)}}{v _2} = 0$$ $$\frac{\partial }{{\partial {\alpha _2}}}\psi ({\alpha _2}) = {\alpha _2}(k(1,1) + k(1,2) - 2k(1,2)) - \zeta {y^{(2)}}k(1,1) + \zeta {y^{(2)}}k(1,2) + {y^{(1)}}{y^{(2)}} - {y^{(1)}}{y^{(2)}} - {y^{(1)}}{v _1} + {y^{(2)}}{v _2} = 0$$我们设\(\eta = k(1,1) + k(1,2) - 2k(1,2)\),对\(\eta\)大于0的情况,导数为0的极值点就是极小值。对于\(\eta\)小于等于0的情况,最小值点肯定取自于边界,我们需要比较函数在\(L\)和\(H\)的大小。
让我们继续简化上面的式子,对于\(\eta\)大于0的情况下,取得极值。由于我们轻易得到下面的关系。
$${v_1} = \sum\limits _{i = 1}^m {{\alpha _i}{y^{(2)}}k(1,i)} + b - b - \sum\limits _{i = 1}^2 {{\alpha _i}{y^{(2)}}k(1,i)} = f({x^{(1)}}) - b - \sum\limits _{i = 1}^2 {{\alpha _i}{y^{(2)}}k(1,i)} $$ $${v_2} = \sum\limits _{i = 1}^m {{\alpha _i}{y^{(2)}}k(2,i)} + b - b - \sum\limits _{i = 1}^2 {{\alpha _i}{y^{(2)}}k(2,i)} = f({x^{(2)}}) - b - \sum\limits _{i = 1}^2 {{\alpha _i}{y^{(2)}}k(2,i)} $$将上面的关系代入的如下极值:
$$\alpha _2^{new} = \frac{{\zeta {y^{(2)}}k(1,1) - \zeta {y^{(2)}}k(1,2) + {y^{(2)}}({y^{(1)}} - f({x^{(1)}}) - ({y^{(2)}} - f({x^{(2)}})) - {y^{(2)}}\sum\limits _{i = 1}^2 {\alpha _i^{old}{y^{(2)}}k(1,i)} + {y^{(2)}}\sum\limits _{i = 1}^2 {\alpha _i^{old}{y^{(2)}}k(2,i)} }}{\eta }$$我们将\(\zeta = {\alpha _1}{y^{(1)}} + {\alpha _2}{y^{(2)}}\)代入上式,得:
$$\alpha _2^{new} = \frac{{{\alpha _1}{y^{(1)}}{y^{(2)}}k(1,1) + {\alpha _2}k(1,1) - {\alpha _1}{y^{(1)}}{y^{(2)}}k(1,2) - {\alpha _2}k(1,2) - {\alpha _1}{y^{(1)}}{y^{(2)}}k(1,1) - {\alpha _2}k(1,2) + {\alpha _2}{y^{(1)}}{y^{(2)}}k(1,2) + {\alpha _2}k(2,2) + {y^{(2)}}({y^{(1)}} - f({x^{(1)}}) - ({y^{(2)}} - f({x^{(2)}}))}}{\eta }$$约掉部分选项,有:
$$\alpha _2^{new} = \alpha _2^{old} + \frac{{{y^{(2)}}({y^{(1)}} - f({x^{(1)}}) - ({y^{(2)}} - f({x^{(2)}})))}}{\eta }$$ $$\alpha _2^{new} = \alpha _2^{old} + \frac{{{y^{(2)}}({e _1} - {e _2})}}{\eta }$$接下来就只剩下求\(b\)的问题了,根据上一节最后转化的KKT条件。对\(0 \leqslant {\alpha _1} \leqslant C\)的情况下,有\({y^{(1)}}({w^T}{x^{(1)}} + b) = 1\)。所以有:
$$b = {y^{(1)}} - \sum\limits _{i = 1}^m {{\alpha _i}} {y^{(i)}}k(1,i)$$进一步展开有:
$$b = {y^{(1)}} - \sum\limits _{i = 3}^m {\alpha _i^{new}{y^{(i)}}k(1,i)} - \alpha _1^{new}{y^{(1)}}k(1,1) - \alpha _2^{new}{y^{(2)}}k(1,2)$$我们知道上面的式子中\({\alpha _3}\)到\({\alpha _m}\)并没有发生变化,因此有:
$$\sum\limits _{i = 3}^m {\alpha _i^{new}{y^{(i)}}k(1,i)} = \sum\limits _{i = 3}^m {\alpha _i^{old}{y^{(i)}}k(1,i)} = \sum\limits _{i = 1}^m {\alpha _i^{old}{y^{(i)}}k(1,i)} - \alpha _1^{old}{y^{(1)}}k(1,1) - \alpha _2^{old}{y^{(2)}}k(1,2) = f({x^{(1)}}) - b - \alpha _1^{old}{y^{(1)}}k(1,1) - \alpha _2^{old}{y^{(2)}}k(1,2)$$代入如上式得:
$${b^{new}} = - {e_1} + (\alpha _1^{old} - \alpha _1^{new}){y^{(1)}}k(1,1) + (\alpha _1^{old} - \alpha _1^{new}){y^{(2)}}k(1,2) + {b^{old}}$$对于\({\alpha _2}\)有同样的道理。
综上,假如\(0 \leqslant {\alpha _1} \leqslant C\),有:
$${b^{new}} = - {e_1} + (\alpha _1^{old} - \alpha _1^{new}){y^{(1)}}k(1,1) + (\alpha _1^{old} - \alpha _1^{new}){y^{(2)}}k(1,2) + {b^{old}}$$假如\(0 \leqslant {\alpha _2} \leqslant C\),有:
$${b^{new}} = - {e_2} + (\alpha _2^{old} - \alpha _2^{new}){y^{(1)}}k(2,1) + (\alpha _2^{old} - \alpha _2^{new}){y^{(2)}}k(2,2) + {b^{old}}$$假如\(0 \leqslant {\alpha _1},{\alpha _2} \leqslant C\),事实上上面两个公司的出来的结果是一样的,因此不用特殊计算。
如果不满足在\(0\)和\(C\)的范围,则去两个公式的中间值。(笔者认为没有必要更新)
8 SMO算法实践
在SMO论文中有具体的伪代码,算法的主要逻辑就是要保证每个样本都满足KKT条件,且直到所有\(\alpha \)达到极值,即不需要更新为止。
然后是关于\(\alpha \)的选择,第一个\(\alpha \)我们可以随机选择一个违反KKT条件的,第二个我们选择能够最大程度更新\(\alpha \)的值,看上一节的公式,实际会选择\(|e _1-e _2|\)最大的样本点作为第二个\(\alpha \)。具体逻辑可以参考SMO的论文,或者下面代码的注释。代码如下:
1 | import numpy as np |
经过数轮迭代得到如下结果,其中直线为得到的分割曲线,蓝色点为支持向量,红色点是那些有良好分类的样本,绿色点为可容忍的误差样本。
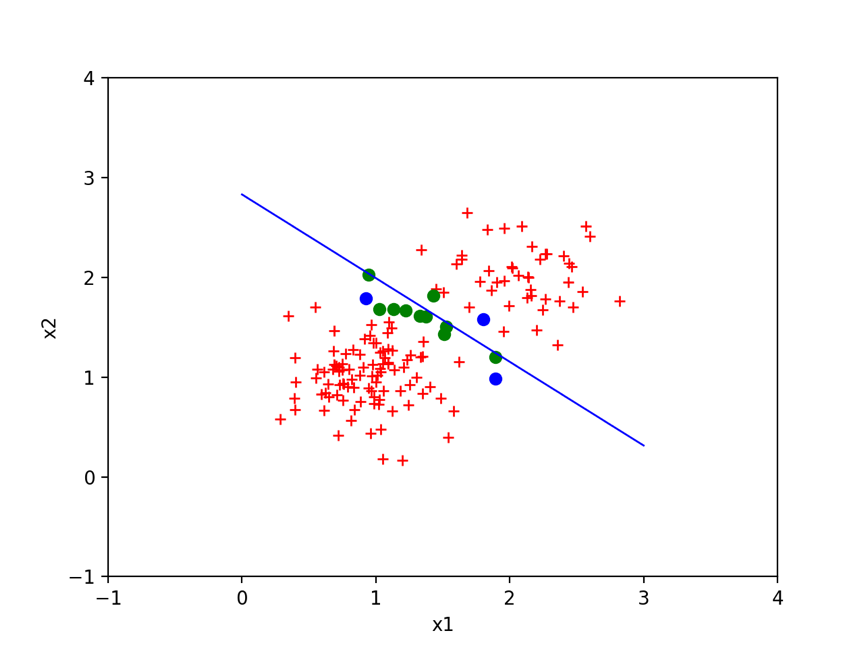
9 核函数
SMO另个非常强大的地方上,它能够很好的解决非线性问题。我们之前的公式中有\(<{x _i},{x _j}>\),他是两个向量的內积,代表着两个样本的相关性。我们把这个叫做核函数。核函数不仅仅是可以为简单的內积,还可以对样本进行多维展开,映射到高维地址空间。这样在低维地址空间线性不可分的样本,在高维空间就变得线性可分了。譬如,\(|x| < 1\)不是线性可分的,但是对其进行\(x \to (x,{x^2})\)映射后,也就的到一个二次曲线,我们可以使用\(y = 1\)进行线性分割。
下面直接引入高斯核函数,它可以对函数进行无线维空间的映射。具体定义如下:
$$k(x,z) = \exp ( - \frac{{||x - z|{|^2}}}{{2{\sigma ^2}}})$$对于核函数的数学理论和几何意义,以及高斯核为啥可以向无限维空间映射,之后有时间需要详细研究。
我们制造一组\(x _1^2 + x _2^2 = 1\)分割的样本,然后尝试对其进行分割,实际上与上一节程序的区别仅仅在于核函数的选取,这里我们使用高斯核函数。
1 | import numpy as np |
经过数轮迭代之后,得到参数。然后在随机产生一些样本,通过训练集得到参数对随机测试样本进行分割,结果如下,发现分割效果还是很理想的。
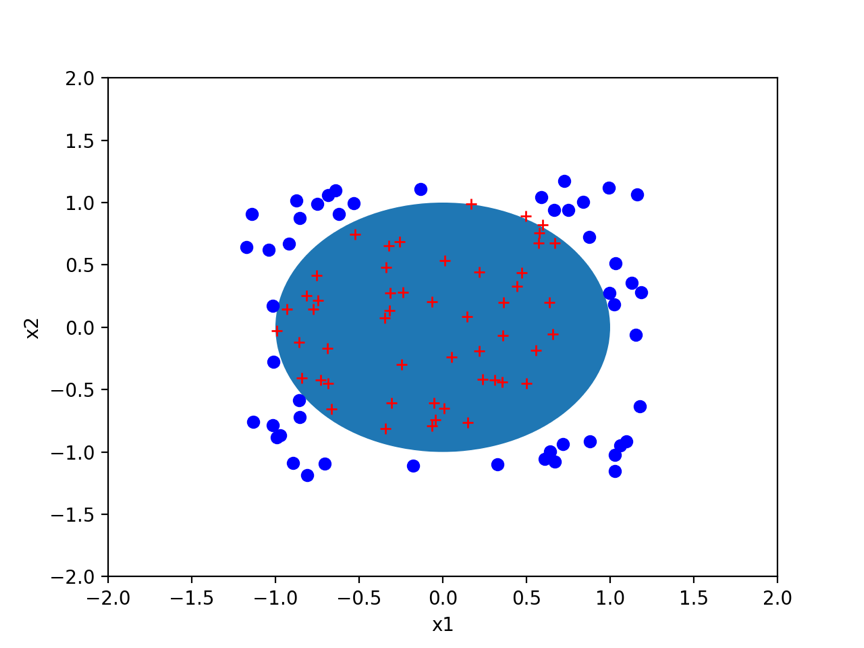
附录
手写公式和word版博客地址:
1 | https://github.com/zhengchenyu/MyBlog/tree/master/doc/mlearning/支持向量机 |
参考文献
- cs229-notes3
- 机器学习 周志华版
- Fast Training of Support Vector Machines Using Sequential Minimal Optimization
- http://blog.csdn.net/luoshixian099/article/details/51227754
机器学习-2.6-监督学习之朴素贝叶斯算法
朴素贝叶斯算法
1 朴素贝叶斯算法
1.1 垃圾邮件处理模型
以预测垃圾邮件为例子,介绍贝叶斯算法。词库中有5000个单词。我们有输入\(x\),\(x \in {\{ 0,1\} ^{50000}}\),是一个50000维的向量,其中\(x _i\)表示词库中第\(i\)个单次在该邮件中出现,为\(0\)表示词库中第\(i\)个单次在该邮件中没有出现。
已经邮件是否为邮件,样本\(({x _1},{x _2},…,{x _i})\)出现的概率为\(p({x _1},{x _2},…,{x _i}|y)\),可以定义为下式:
$$p({x _1},{x _2},...,{x _i}|y) = p({x _1}|y)p({x _2}|y,{x _1})...p({x _{50000}}|y,{x _1},...,{x _{49999}})$$注: 上式很好理解。右侧第一个式子为已知\(y\),\(x _1\)出现的概率。第二个为一直\(y\)和\(x _1\)出现\(x _2\)的概率。因此两个式子相乘表示一直\(y\)初选\((x1,x2)\)的概率。依次类推,可以得到这个公式。
$$p({x _1},{x _2},...,{x _i}|y) = p({x _1}|y)p({x _2}|y)...p({x _{50000}}|y) = \prod\limits _{i = 1}^{50000} {p({x _i}|y)}$$1.2 公式推导
关于最大释然函数如何设置,我们需要知道我们的目标是找到参数\(\theta\),使得训练时候在给定\(x\)的情况下,得到最准确\(y\)的概率最大,最大释然函数就是这些概率的连乘,具体是需要保证下面的式子最大:
$$\ln \prod\limits _{i = 1}^m {p(y = {y^i}|x = {x^i};\theta )p(x = {x^i})} $$在很多教材中都介绍最大释然函数是如下的表达,实际上是一样的。下面的式子的直接意义是在\(\theta\)已知情况下,出现\((x^i,y^i)\)的概率,实际上面的分析过程是一样的。
$$\ln \prod\limits _{i = 1}^m {p(y = {y^i},x = {x^i};\theta )} $$后面为了简写后面省略了\(\theta\)。
我们做如下设置:
$${\phi _y} = p(y = 1)$$ $${\phi _{i|y = 1}} = p({x _i} = 1|y = 1)$$ $${\phi _{i|y = 0}} = p({x _i} = 1|y = 0)$$然后得到最大释然函数:
$$\ell ({\phi _y},{\phi _{i|y = 1}},{\phi _{i|y = 0}}) = \ln \prod\limits _{i = 1}^m {p(y = {y^i},x = {x^i})} = \ln \prod\limits _{i = 1}^m {p({x^i}|{y^i})p({y^i})}$$ $$\ell ({\phi _y},{\phi _{i|y = 1}},{\phi _{i|y = 0}}) = \ln \prod\limits _{i = 1}^m {[[1\{ {y^i} = 1\} \ln ((\prod\limits _{j = 1}^n {p(x _j^i|{y^i})p({y^i} = 1)} ){\phi _y})][1\{ {y^i} = 1\} \ln ((\prod\limits _{j = 1}^n {p(x _j^i|{y^i})p({y^i} = 0)} )(1 - {\phi _y}))]} ]$$ $$\ell ({\phi _y},{\phi _{i|y = 1}},{\phi _{i|y = 0}}) = \sum\limits _{i = 1}^m {[[1\{ {y^i} = 1\} (\ln {\phi _y} + \ln \prod\limits _{j = 1}^n {({{({\phi _{j|y = 1}})}^{1\{ {x _j} = 1\} }}{{(1 - {\phi _{j|y = 1}})}^{1\{ {x _j} = 0\} }})} )][1\{ {y^i} = 0\} (\ln (1 - {\phi _y}) + \ln \prod\limits _{j = 1}^n {({{({\phi _{j|y = 0}})}^{1\{ {x _j} = 1\} }}{{(1 - {\phi _{j|y = 0}})}^{1\{ {x _j} = 0\} }})} )]]}$$ $$\ell ({\phi _y},{\phi _{i|y = 1}},{\phi _{i|y = 0}}) = \sum\limits _{i = 1}^m {[[1\{ {y^i} = 1\} (\ln {\phi _y} + \sum\limits _{j = 1}^n {(1\{ x _j^i = 1\} \ln {\phi _{j|y = 1}} + 1\{ x _j^i = 0\} \ln (1 - {\phi _{j|y = 1}}))} )][1\{ {y^i} = 0\} (\ln (1 - {\phi _y}) + \sum\limits _{j = 1}^n {(1\{ x _j^i = 1\} \ln {\phi _{j|y = 0}} + 1\{ x _j^i = 0\} \ln (1 - {\phi _{j|y = 0}}))} )]]}$$然后我们对\(\phi _y\)求偏导数:
$$\frac{{\partial \ell ({\phi _y},{\phi _{i|y = 1}},{\phi _{i|y = 0}})}}{\partial {\phi _y}} = \sum\limits _{i = 1}^m {(1\{ {y^i} = 1\} \frac{1}{\phi _y} + 1\{ {y^i} = 0\} \frac{ - 1}{1 - {\phi _y}})} = \sum\limits _{i = 1}^m {\frac{1\{ {y^i} = 1\} - {\phi _y}}{\phi _y}(1 - {\phi _y})} = 0$$所以有:
$${\phi _y} = \frac{1}{m}\sum\limits _{i = 1}^m {1\{ {y^i} = 1\} }$$然后继续求偏导数:
$$\frac{{\partial \ell ({\phi _y},{\phi _{i|y = 1}},{\phi _{i|y = 0}})}}{{\partial {\phi _{i|y = 1}}}} = \sum\limits _{i = 1}^m {1\{ {y^i} = 1\} (1\{ x _j^i = 1\} \frac{1}{{{\phi _{x _j^i = 1|y = 1}}}} + 1\{ x _j^i = 0\} \frac{{ - 1}}{{1 - {\phi _{x _j^i = 1|y = 1}}}})}=0$$ $$\frac{{\partial \ell ({\phi _y},{\phi _{i|y = 1}},{\phi _{i|y = 0}})}}{{\partial {\phi _{i|y = 1}}}} = \sum\limits _{i = 1}^m {1\{ {y^i} = 1\} (\frac{{1\{ x _j^i = 1\} - {\phi _{i|y = 1}}}}{{{\phi _{i|y = 1}}(1 - {\phi _{i|y = 1}})}})}=0$$如果一个单词不再训练样本中,就会出现0/0的现象。避免这个事件发生,引入拉普拉斯平滑。所以有:
$${\phi _{i|y = 1}} = \frac{{\sum\limits _{i = 1}^m {1\{ x _j^i = 1,{y^i} = 1\} } + 1}}{{\sum\limits _{i = 1}^m {1\{ {y^i} = 1\} } + 2}}$$同理有:
$${\phi _{i|y = 0}} = \frac{{\sum\limits _{i = 1}^m {1\{ x _j^i = 1,{y^i} = 0\} } + 1}}{{\sum\limits _{i = 1}^m {1\{ {y^i} = 1\} } + 2}}$$注:试分析这样的公式,\(\phi _y\)就是总的垃圾邮件数目除以从样本数。\({\phi _{i|y = 1}}\)就是所有垃圾邮件中出现\(x _j^i\)对应的单词的数目除以所有垃圾邮件的数目。实际上这是个很容理解的道理。当然这就是数学的魅力,即便很简单的公司都是有着其理论依据的。
1.3 算法实现
我们使用样本得到各个单词的概率分布,然后预测邮件是否为垃圾邮件。运行下面的程序,可以得到了正确的垃圾邮件分类。
1 | import numpy as np |
2 多元伯努利事件模型
2.1 公式推导
下面采用另外一种方式进行建模。对于\({x^T} = [{x _1},{x _2},…,{x _n}]\),其中\({x _1} = 1\)代表邮件的第一个单词在词库中的索引为1。仍然有如下公式:
$$p({x _1},{x _2},...,{x _i}|y) = \prod\limits _{i = 1}^n {p({x _i}|y)}$$我们设置\({\phi _y} = p(y = 1)\),\({\phi _{i = k|y = 1}} = p({x _i} = k|y = 1)\)。
然后求最大释然函数:
$$\ell ({\phi _y},{\phi _{i = k|y = 1}},{\phi _{i = k|y = 0}}) = \ln \prod\limits _{i = 1}^m {p({y^i}|{x^i})} = \ln \prod\limits _{i = 1}^m {p({x^i}|{y^i})p({y^i})}$$ $$\ell ({\phi _y},{\phi _{i = k|y = 1}},{\phi _{i = k|y = 0}}) = \ln \prod\limits _{i = 1}^m {{{(p({x^i}|{y^i} = 1){\phi _y})}^{1\{ {y^i} = 1\} }}p({x^i}|{y^i} = 0)(1 - {\phi _y}){)^{1\{ {y^i} = 0\} }})}$$ $$\ell ({\phi _y},{\phi _{i = k|y = 1}},{\phi _{i = k|y = 0}}) = \ln \prod\limits _{i = 1}^m {{{((\ln (\prod\limits _{j = 1}^n {p(} x _j^i|{y^i} = 1)){\phi _y})}^{1\{ {y^i} = 1\} }}(\ln (\prod\limits _{j = 1}^n {p(} x _j^i|{y^i} = 0))(1 - {\phi _y}){)^{1\{ {y^i} = 0\} }})}$$ $$\ell ({\phi _y},{\phi _{i = k|y = 1}},{\phi _{i = k|y = 0}}) = \sum\limits _{i = 1}^m {[1\{ {y^i} = 1\} (\ln {\phi _y} + \sum\limits _{j = 1}^n {\ln p(x _j^i|{y^i} = 1)} ) + 1\{ {y^i} = 0\} (\ln (1 - {\phi _y}) + \sum\limits _{j = 1}^n {\ln p(x _j^i|{y^i} = 0)} )]}$$我们对\({\phi _y}\)求偏导数,与之前相同,这里直接写出:
$${\phi _y} = \frac{1}{m}\sum\limits _{i = 1}^m {1\{ {y^i} = 1\} }$$我们对\({\phi _{i = k|y = 0}}\)求偏导数,如下:
$$\frac{{\partial \ell ({\phi _y},{\phi _{i = k|y = 1}},{\phi _{i = k|y = 0}})}}{{\partial {\phi _{i = k|y = 1}}}} = \frac{{\partial \sum\limits _{i = 1}^m {1\{ {y^i} = 1\} \sum\limits _{j = 1}^n {\ln p({x^i}|{y^i} = 1)} } }}{{\partial {\phi _{i|y = 1}}}}$$其中有:
$$\ln p({x^i}|{y^i} = 1) = \ln [p{(x _j^i = k|{y^i} = 1)^{1\{ x _j^i = k\} }}p{(x _j^i \ne k|{y^i} = 1)^{1\{ x _j^i \ne k\} }}]$$ $$\ln p({x^i}|{y^i} = 1) = 1\{ x _j^i = k\} \ln {\phi _{i = k|y = 1}} + (1 - 1\{ x _j^i = k\} )\ln (1 - {\phi _{i = k|y = 1}})$$所以有:
$$\frac{{\partial \ell ({\phi _y},{\phi _{i = k|y = 1}},{\phi _{i = k|y = 0}})}}{{\partial {\phi _{i = k|y = 1}}}} = \sum\limits _{i = 1}^m {1\{ {y^i} = 1\} \sum\limits _{j = 1}^n {\frac{{1\{ x _j^i = k\} - {\phi _{i = k|y = 1}}}}{{{\phi _{i = k|y = 1}}(1 - {\phi _{i = k|y = 1}})}}} } = 0$$根据拉普拉斯平滑,所以最后得到下面的公式,其中V为词库中单词的数目。
$${\phi _{i = k|y = 1}} = \frac{{\sum\limits _{i = 1}^m {\sum\limits _{j = 1}^n {1\{ {y^i} = 1,x _j^i = k\} } } + 1}}{{n\sum\limits _{i = 1}^m {1\{ {y^i} = 1\} } + V}}$$同理有:
$${\phi _{i = k|y = 0}} = \frac{{\sum\limits _{i = 1}^m {\sum\limits _{j = 1}^n {1\{ {y^i} = 0,x _j^i = k\} } } + 1}}{{n\sum\limits _{i = 1}^m {1\{ {y^i} = 0\} } + V}}$$2.2 算法的实现
我们使用样本得到各个单词的概率分布,然后预测邮件是否为垃圾邮件。运行下面的程序,可以得到了正确的垃圾邮件分类。
1 | import numpy as np |
由于概率值过小,多次乘积会超过浮点数精度范围,所以程序这里乘以一个固定的比例系数
附录
手写公式和word版博客地址:
1 | https://github.com/zhengchenyu/MyBlog/tree/master/doc/mlearning/贝叶斯算法 |
机器学习-2.5-监督学习之生成型学习算法
生成型学习算法
1 生成型学习算法简述
我们之前讲到的算法。譬如逻辑回归,试图从\(x\)直接学习得到一组映射关系到\(y\),即通过样本\((x,y)\)学习得到,使得其能够准确的预测\(y\)。这类算法叫做判别性学习算法。
本节我们将介绍生成学习型算法。举个例子,对于一个大象,我们可以为大象构造一个关于其特征的模型。对于一条狗,同样可以为狗构造一个关于关于其特征的模型。对于一个新动物,我们可以根据前述构建的模型,判断这个动物是像大象多一点还是像狗多一点,来确定这动物是大象还是狗。
我们可以根据样本得到大象的特征模型\(p(x|y=0)\)和狗的特征模型\(p(x|y=1)\),同时也可以得到\(p(y)\)。根据贝叶斯公式,有如下:
$$\max (p(y|x)) = \max (\frac{p(x|y)p(y)}{p(x)}) = \max (\frac{p(x|y)p(y)}{p(x|y = 1)p(y = 1) + p(x|y = 0)p(y = 0)})$$对于我们做预测的时候,可以不考虑分母。
$$\max (p(y|x)) = \max (p(x|y)p(y))$$为什么不考虑分母?我们可以将\(p(x|y)p(y)\)简化为\(f(y)\),因此分母就是\(f(1)+f(0)\)。如果我们通过样本对完成了建模,因此分母就是一个常数了,因此没有计算的必要了。
2 高斯判别分析
2.1 多维高斯分布介绍
略,详见cs229-note2.pdf
2.2 高斯判别分析模型
对于一个分类问题,\(y \in (0,1)\)。假设\(x\)是连续的随机变量,服从高斯分布。具体描述如下:
$$y \sim Bernoulli(\phi)$$ $$x|y = 0 \sim N({\mu _0},\Sigma)$$ $$x|y = 1 \sim N({\mu _1},\Sigma)$$ $$p(x|y = 0) = \frac{1}{{{(2\pi )}^{\frac{n}{2}}}|\Sigma {|^{\frac{n}{2}}}}\exp ( - \frac{1}{2}{(x - {\mu _0})^T}{\Sigma ^{ - 1}}(x - {\mu _0}))$$ $$p(x|y = 1) = \frac{1}{{{(2\pi )}^{\frac{n}{2}}}|\Sigma {|^{\frac{n}{2}}}}\exp ( - \frac{1}{2}{(x - {\mu _1})^T}{\Sigma ^{ - 1}}(x - {\mu _1}))$$2.3 公式推导
我们设置\(\phi = p(y = 0)\)。根据前面的分析,在给定样本的情况下,我们需要保证下式最大。
$$\prod\limits _{i = 1}^m {p({y^i}|{x^i})} = \prod\limits _{i = 1}^m {p({x^i}|{y^i})p({y^i})}$$这里为了简写,我们设置\({z _0} = x - {\mu _0}\) ,\({z _1} = x - {\mu _1}\)。取自然对数后,得到最大释然函数:
$$\ell ({\mu _1},{\mu _1},\Sigma ,\phi ) = \sum\limits _{i = 1}^m {\ln \{ {{[\frac{1}{{{{(2\pi )}^{\frac{n}{2}}}|\Sigma {|^{\frac{n}{2}}}}}\exp ( - \frac{1}{2}z _0^T{\Sigma ^{ - 1}}{z _0})]}^{1\{ {y^i} = 0\} }}{{[\frac{1}{{{{(2\pi )}^{\frac{n}{2}}}|\Sigma {|^{\frac{n}{2}}}}}\exp ( - \frac{1}{2}z _1^T{\Sigma ^{ - 1}}{z _1})]}^{1\{ {y^i} = 1\} }}\} } $$ $$\ell ({\mu _0},{\mu _1},\Sigma ,\phi ) = \sum\limits _{i = 1}^m {\{ 1\{ {y^i} = 0\} [ - \frac{1}{2}z _0^T{\Sigma ^{ - 1}}{z _0} - \frac{n}{2}\ln 2\pi - \frac{n}{2}\ln |\Sigma | + \ln \phi ] + 1\{ {y^i} = 1\} } [ - \frac{1}{2}z _1^T{\Sigma ^{ - 1}}{z _1} - \frac{n}{2}\ln 2\pi - \frac{n}{2}\ln |\Sigma | + \ln (1 - \phi )]\}$$然后我们分别对各个参数求偏导数:
$$\frac{{\partial \ell ({\mu _0},{\mu _1},\sum ,\phi )}}{{\partial \phi }} = \sum\limits _{i = 1}^m {(\frac{{1\{ {y^i} = 1\} }}{\phi } - \frac{{1\{ {y^i} = 0\} }}{{1 - \phi }})} = \sum\limits _{i = 1}^n {(\frac{{1\{ {y^i} = 1\} }}{\phi } - \frac{{1 - 1\{ {y^i} = 1\} }}{{1 - \phi }})} = 0$$所以有:
$$\phi = \frac{1}{m}\sum\limits _{i = 1}^n {1\{ {y^i} = 1\} }$$然后对\(\mu _0\)求导:
$${\nabla _{{\mu _0}}}\ell ({\mu _0},{\mu _1},\Sigma ,\phi ) = \sum\limits _{i = 1}^m {{\nabla _{{\mu _0}}}( - \frac{1}{2}{{({x^i} - {\mu _0})}^T}{\Sigma ^{ - 1}}({x^i} - {\mu _0}))1\{ {y^i} = 0\} }$$ $${\nabla _{{\mu _0}}}\ell ({\mu _0},{\mu _1},\Sigma ,\phi ) = - \frac{1}{2}\sum\limits _{i = 1}^m {{\nabla _{{\mu _0}}}( - \mu _0^T{\Sigma ^{ - 1}}{x^i} - {{({x^i})}^T}{\Sigma ^{ - 1}}{\mu _0} + \mu _0^T{\Sigma ^{ - 1}}{\mu _0})1\{ {y^i} = 0\} }$$我们对上面的式子分头计算。
$${\nabla _{{\mu _0}}}\mu _0^T{\Sigma ^{ - 1}}x = {\nabla _{{\mu _0}}}tr(\mu _0^T{\Sigma ^{ - 1}}{x^i}) = {[{\nabla _{\mu _0^T}}tr(\mu _0^T{\Sigma ^{ - 1}}{x^i})]^T} = {[{({\Sigma ^{ - 1}}{x^i})^T}]^T} = {\Sigma ^{ - 1}}{x^i}$$ $${\nabla _{{\mu _0}}}{({x^i})^T}{\Sigma ^{ - 1}}{\mu _0} = {\nabla _{{\mu _0}}}tr({({x^i})^T}{\Sigma ^{ - 1}}{\mu _0}) = {\nabla _{{\mu _0}}}{\mu _0}{({x^i})^T}{\Sigma ^{ - 1}} = {({({x^i})^T}{\Sigma ^{ - 1}})^T} = {\Sigma ^{ - 1}}{x^i}$$ $${\nabla _{{\mu _0}}}\mu _0^T{\Sigma ^{ - 1}}{\mu _0} = {[{\nabla _{\mu _0^T}}\mu _0^T{\Sigma ^{ - 1}}{\mu _0}E]^T} = [E\mu _0^T{\Sigma ^{ - 1}} + {E^T}\mu _0^T{({\Sigma ^{ - 1}})^T}] = 2{\Sigma ^{ - 1}}{\mu _0}$$\(\Sigma\)为对角阵。由于求偏微分的数为一个常数,因此该值与其迹的相同。另外,这里使用了一些关于迹的公式。具体详见附录。
所以有:
$${\nabla _{{\mu _0}}}\ell ({\mu _0},{\mu _1},\Sigma ,\phi ) = - \sum\limits _{i = 1}^m {{\nabla _{{\mu _0}}}({\Sigma ^{ - 1}}{\mu _0} - {\Sigma ^{ - 1}}{x^i})1\{ {y^i} = 0\} } = 0$$ $${\mu _0} = \frac{{\sum\limits _{i = 1}^m {1\{ {y^i} = 0\} {x^i}} }}{{\sum\limits _{i = 1}^m {1\{ {y^i} = 0\} } }}$$同理有:
$${\mu _1} = \frac{{\sum\limits _{i = 1}^m {1\{ {y^i} = 1\} {x^i}} }}{{\sum\limits _{i = 1}^m {1\{ {y^i} = 1\} } }}$$然后对\(\Sigma^{-1}\)求偏导数。
$${\nabla _{{\Sigma ^{ - 1}}}}\ell ({\mu _0},{\mu _1},\Sigma ,\phi ) = \sum\limits _{i = 1}^m {{\nabla _{{\Sigma ^{ - 1}}}}[( - \frac{1}{2}z _0^T{\Sigma ^{ - 1}}{z _0} + \frac{1}{2}\ln \frac{1}{{|\Sigma |}})1\{ {y^i} = 0\} + ( - \frac{1}{2}z _1^T{\Sigma ^{ - 1}}{z _1} + \frac{1}{2}\ln \frac{1}{{|\Sigma |}})1\{ {y^i} = 1\} ]}$$这里分开计算:
$${\nabla _{\Sigma ^{ - 1}}}(z _0^T{\Sigma ^{ - 1}}{z _0}) = {\nabla _{\sum ^{ - 1}}}tr(z _0^T{\Sigma ^{ - 1}}{z _0}) = {\nabla _{\sum ^{ - 1}}}tr({\Sigma ^{ - 1}}{z _0}z _0^T) = {({z _0}z _0^T)^T} = {z _0}z _0^T$$ $${\nabla _{\Sigma ^{ - 1}}}\ln \frac{1}{|\Sigma |} = {\nabla _{\Sigma ^{ - 1}}}\ln |{\Sigma ^{ - 1}}| = \frac{1}{|{\Sigma ^{ - 1}}|}{\nabla _{\Sigma ^{ - 1}}}{\Sigma ^{ - 1}} = {({({\Sigma ^{ - 1}})^{ - 1}})^T}$$所以有:
$${\nabla _{{\sum ^{ - 1}}}}\ell ({\mu _0},{\mu _1},\Sigma ,\phi ) = \sum\limits _{i = 1}^m {[( - \frac{1}{2}{z _0}z _0^T + \frac{1}{2}\Sigma )1\{ {y^i} = 0\} + ( - \frac{1}{2}{z _1}z _1^T + \frac{1}{2}\Sigma )1\{ {y^i} = 1\} ]} = 0$$ $$\Sigma = \frac{1}{m}\sum\limits _{i = 1}^m {[({x^i} - {\mu _0}){{({x^i} - {\mu _0})}^T}1\{ {y^i} = 0\} + ({x^i} - {\mu _1}){{({x^i} - {\mu _1})}^T}1\{ {y^i} = 1\} ]}$$ $$\Sigma = \frac{1}{m}\sum\limits _{i = 1}^m {[({x^i} - {\mu _{{y^i}}}){{({x^i} - {\mu _{{y^i}}})}^T}]}$$2.3 高斯型算法实例
我们分别以 \((1,1)\)和\((2,2)\)为均值生成一组高斯分布。下面图是生成的样本。
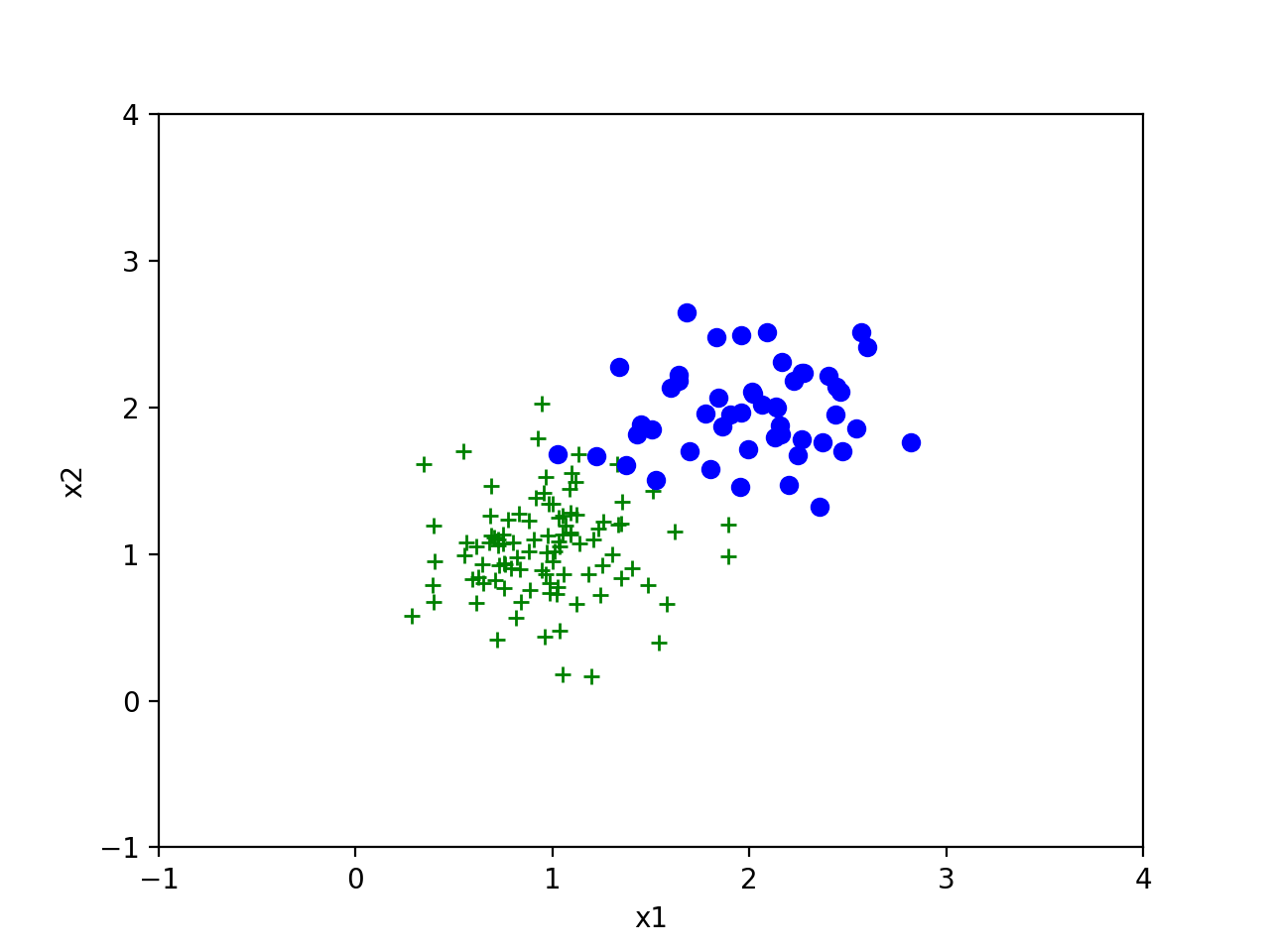
前提假设是我们知道两组分类是符合高斯分布的,切假定协方差相同。但我们不知道两组数据的均值和协方差。根据前面的公式有如下代码:
1 | import numpy as np |
对于两个协方差相同的样本,我们知道两组数据有相同的概率分布曲线,具体都应该是一个个同心圆。因此,我们可以确定一条垂直于两点连线的曲线是对该样本的理论上正确的分割。因此,我们得到如下结果,并与理论分割的曲线,即\(y=-x+3\)比较,发现对我们随机产生的样本分类效果非常好。
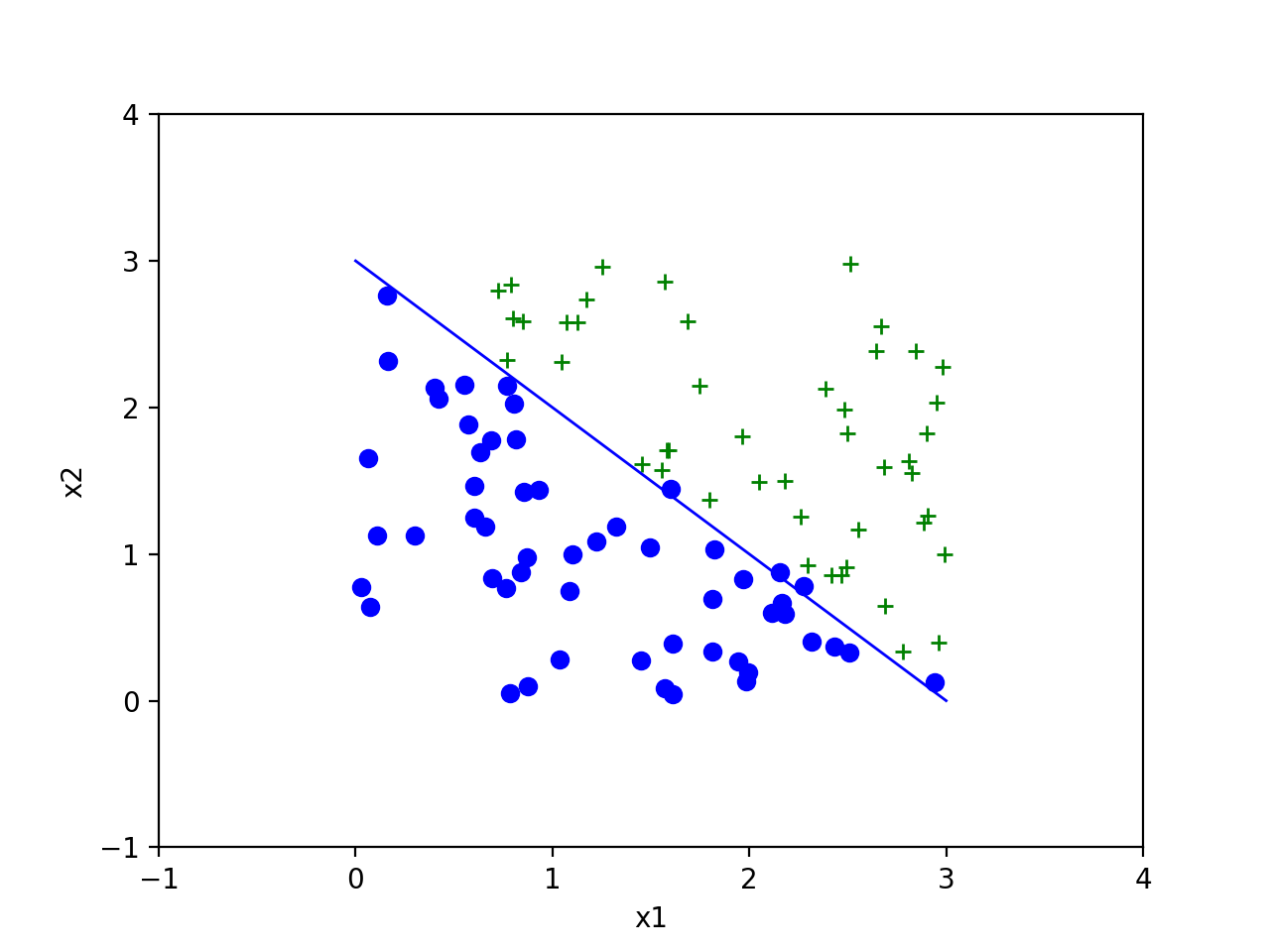
附录 A 相关公式
Hessian矩阵定义:
$${\nabla _A}f(A) = \left( {\begin{array}{*{20}{c}} {\frac{{\partial f}}{{\partial {A _{11}}}}}& \ldots &{\frac{{\partial f}}{{\partial {A _{1n}}}}} \\\ \vdots & \ddots & \vdots \\\ {\frac{{\partial f}}{{\partial {A _{n1}}}}}& \cdots &{\frac{{\partial f}}{{\partial {A _{nn}}}}} \end{array}} \right)$$矩阵的迹的定义:
$$trA = \sum\limits _{i = 1}^n {{A _{ii}}}$$关于矩阵迹的公式:
$$tr(a) = a$$ $$tr(aA) = atr(A)$$ $$tr(aA) = atr(A)$$ $$tr(ABC) = tr(CAB) + tr(BCA)$$ $$trA = tr{A^T}$$ $$tr(A + B) = trA + trB$$ $${\nabla _A}tr(AB) = {B^T}$$ $${\nabla _{A^T}}f(A) = {({\nabla _A}f(A))^T}$$ $${\nabla _{A^T}}trAB{A^T}C = CAB + {C^T}A{B^T}$$ $${\nabla _{A^T}}trAB{A^T}C = CAB + {C^T}A{B^T}$$对矩阵做展开能证明上述大部分公式,这里暂时略。
附录B
手写公式和word版博客地址:
1 | https://github.com/zhengchenyu/MyBlog/tree/master/doc/mlearning/生成型学习算法 |